An electrocardiogram classifying method based on a deep learning algorithm
A technology of deep learning and classification methods, applied in medical science, sensors, diagnostic recording/measurement, etc., can solve problems such as incorrect calculation parameters and misclassification, and achieve the effect of improving data accuracy, accuracy, and accuracy
- Summary
- Abstract
- Description
- Claims
- Application Information
AI Technical Summary
Problems solved by technology
Method used
Image
Examples
Embodiment 1
[0032] The electrocardiogram classification method based on deep learning algorithm, its flow chart is as follows figure 1 As shown, it specifically includes the following steps:
[0033] a. (1) Obtain single-lead ECG waveform data and ECG additional information, and intercept the data with a length of 10 seconds as the original ECG waveform data according to the single-lead ECG waveform data, wherein the single-lead ECG waveform data can be passed through Some databases, such as the ECG waveform database (CSE), or obtained through other means, ECG additional information includes gender, height, bust, weight, fat percentage, race.
[0034] (2) as required, the original electrocardiogram waveform data obtained in step (1) can be denoised, and the denoised process includes the following steps:
[0035] a11. Use a high-pass filter to remove baseline drift noise;
[0036] a12. Confirm whether the noise is too high based on the standard variance and threshold method of the PQ segme...
Embodiment 2
[0053] The electrocardiogram classification method based on deep learning algorithm, its flow chart is as follows figure 1 As shown, it specifically includes the following steps:
[0054] a. (1) Obtain single-lead ECG waveform data and ECG additional information, and intercept the data with a length of 8 seconds as the original ECG waveform data according to the single-lead ECG waveform data, in which the single-lead ECG waveform data and ECG additional information Information can be obtained through physical examination results, existing databases such as the ECG waveform database (CSE), or other means. Additional information on the ECG includes gender, height, bust, weight, fat percentage, and race .
[0055] (2) as required, the original electrocardiogram waveform data obtained in step (1) can be denoised, and the denoised process includes the following steps:
[0056] a11. Use a high-pass filter to remove baseline drift noise;
[0057] a12. Confirm whether the noise is ...
Embodiment 3
[0074] The electrocardiogram classification method based on deep learning algorithm, its flow chart is as follows figure 1 As shown, it specifically includes the following steps:
[0075] a. (1) Obtain single-lead ECG waveform data and ECG additional information, and intercept the data with a length of 16 seconds as the original ECG waveform data according to the single-lead ECG waveform data, in which the single-lead ECG waveform data and ECG additional information Information can be obtained through physical examination results, existing databases such as the ECG waveform database (CSE), or other means. Additional information on the ECG includes gender, height, bust, weight, fat percentage, and race .
[0076] (2) as required, the original electrocardiogram waveform data obtained in step (1) can be denoised, and the denoised process includes the following steps:
[0077] a11. Use a high-pass filter to remove baseline drift noise;
[0078] a12. Confirm whether the noise is...
PUM
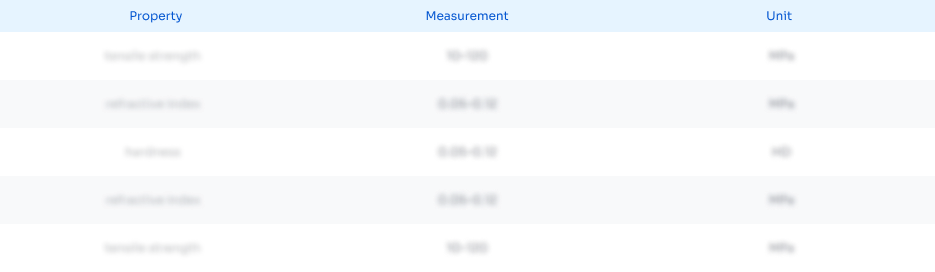
Abstract
Description
Claims
Application Information
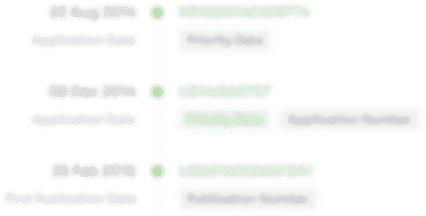
- Generate Ideas
- Intellectual Property
- Life Sciences
- Materials
- Tech Scout
- Unparalleled Data Quality
- Higher Quality Content
- 60% Fewer Hallucinations
Browse by: Latest US Patents, China's latest patents, Technical Efficacy Thesaurus, Application Domain, Technology Topic, Popular Technical Reports.
© 2025 PatSnap. All rights reserved.Legal|Privacy policy|Modern Slavery Act Transparency Statement|Sitemap|About US| Contact US: help@patsnap.com