Sample label missing data classifier training method
A technology with missing data and sample labels, applied in the field of data analysis, can solve problems such as poor generality, poor performance of classifiers or classification methods, etc.
- Summary
- Abstract
- Description
- Claims
- Application Information
AI Technical Summary
Problems solved by technology
Method used
Image
Examples
Embodiment Construction
[0084] The present invention is further described below in conjunction with accompanying drawing and embodiment. Select 3 polypeptide identification datasets to test the effectiveness of the disclosed method. These 3 datasets are listed in Table 1: the total number of samples of yeast, ups1 and tal08; The number of known negative samples; the number of unlabeled samples. Each data set is randomly divided into two sub-sets according to the ratio of 1:1 - training set and test set. The data processing method provided by the present invention is on the training set Compute, get the classification function, and test the performance of the classification function on an independent test set.
[0085] For the three data sets tested, a unified parameter setting is adopted: in the adaptive semi-supervised learning model (8), μ=5.0, c 1 = c 2 = 1.0, adopt the square loss function, and determine it by formula (6) In the termination criterion of Algorithm 1, r 1 The value is 0.5.
[...
PUM
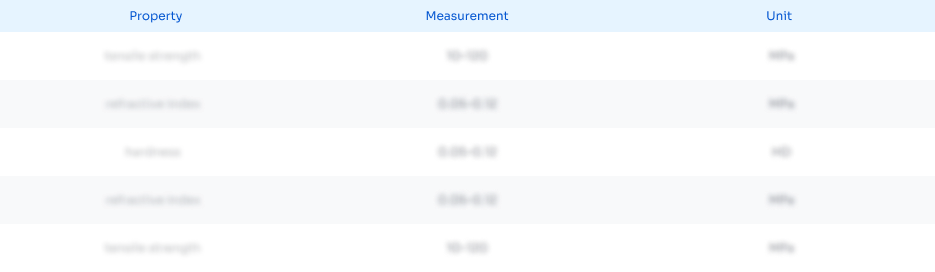
Abstract
Description
Claims
Application Information
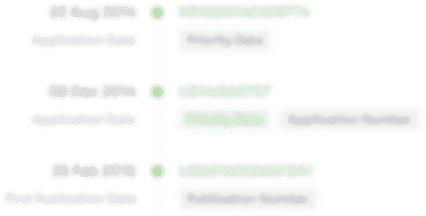
- R&D Engineer
- R&D Manager
- IP Professional
- Industry Leading Data Capabilities
- Powerful AI technology
- Patent DNA Extraction
Browse by: Latest US Patents, China's latest patents, Technical Efficacy Thesaurus, Application Domain, Technology Topic, Popular Technical Reports.
© 2024 PatSnap. All rights reserved.Legal|Privacy policy|Modern Slavery Act Transparency Statement|Sitemap|About US| Contact US: help@patsnap.com