SAR image target discrimination method based on weakly supervised learning
A technology of target identification and weak supervision, applied in the field of target identification, it can solve the problems of time-consuming and labor-intensive, complex clutter data, affecting the learning of the discriminator, etc., to achieve the effect of reducing cost and excellent identification performance.
- Summary
- Abstract
- Description
- Claims
- Application Information
AI Technical Summary
Problems solved by technology
Method used
Image
Examples
Embodiment Construction
[0034] refer to figure 1 , the realization of the present invention is divided into two stages of training and testing, and its steps are as follows:
[0035] 1. Training stage
[0036] Step 1, extract the dense scale-invariant feature transform SIFT feature of each sample in the training sample set.
[0037] (1.1) Input training sample set X={X + ,X -}, where X + Is the sample set of positive images, both positive and negative samples, X - is the negative sample set of the negative image, and all samples are negative samples;
[0038] (1.2) Extract the dense scale-invariant feature transformation SIFT feature of the training sample x in the training sample set:
[0039] (1.2a) Perform two-norm normalization on the training sample x to obtain the normalized training sample: Then set the Gaussian template M with a size of 5×5:
[0040] M = 0.0030 0.0133 0.0219...
PUM
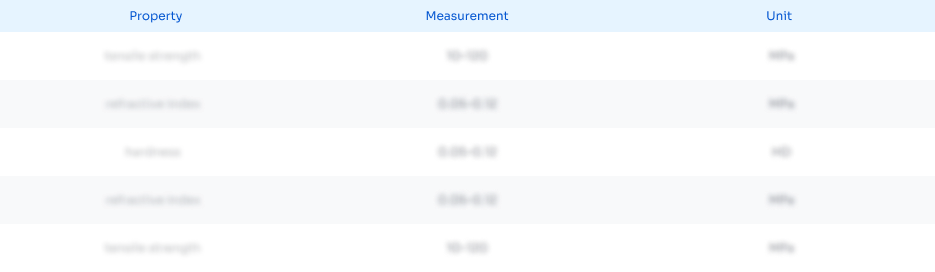
Abstract
Description
Claims
Application Information
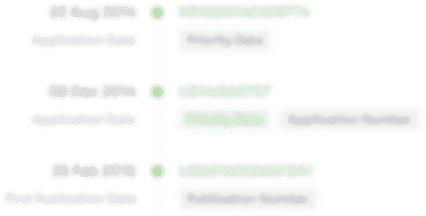
- R&D Engineer
- R&D Manager
- IP Professional
- Industry Leading Data Capabilities
- Powerful AI technology
- Patent DNA Extraction
Browse by: Latest US Patents, China's latest patents, Technical Efficacy Thesaurus, Application Domain, Technology Topic, Popular Technical Reports.
© 2024 PatSnap. All rights reserved.Legal|Privacy policy|Modern Slavery Act Transparency Statement|Sitemap|About US| Contact US: help@patsnap.com