Traffic image retrieval method based on depth learning
A technology of deep learning and image retrieval, which is applied in multi-attribute deep hash coding image retrieval, realizes the field of traffic image retrieval based on deep learning, and can solve the problem of not using attribute feature information, decreasing the accuracy of retrieval, and not being able to use several complexity Features to express and other issues, to achieve accurate and effective video image search
- Summary
- Abstract
- Description
- Claims
- Application Information
AI Technical Summary
Problems solved by technology
Method used
Image
Examples
Embodiment Construction
[0041] The drawings are for illustrative purposes only, and should not be construed as limitations on this patent; in order to better illustrate this embodiment, some parts in the drawings will be omitted, enlarged or reduced, and do not represent the size of the actual product;
[0042] For those skilled in the art, it is understandable that some well-known structures and descriptions thereof may be omitted in the drawings. The technical solutions of the present invention will be further described below in conjunction with the accompanying drawings and embodiments.
[0043] Such asfigure 1 As shown, the method for realizing traffic image retrieval based on deep learning disclosed by the present invention comprises the following steps:
[0044] Step 1: The traffic monitoring video data set used in the example of the present invention comes from the highway monitoring video of Guangzhou University City. After the video is divided into frames, it contains 12510 images of photos...
PUM
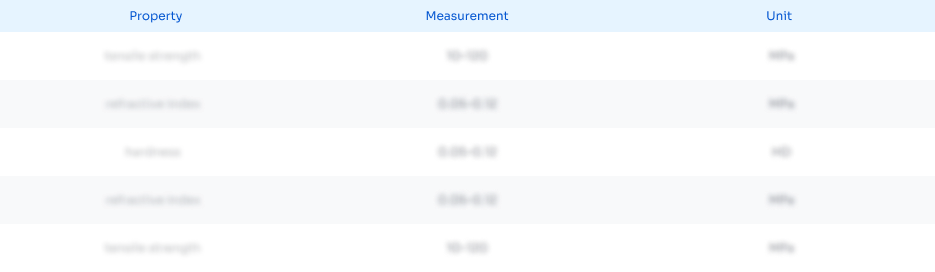
Abstract
Description
Claims
Application Information
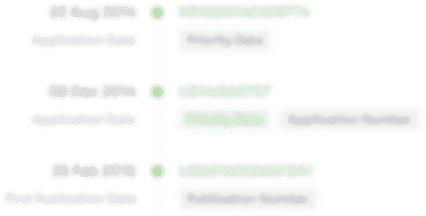
- R&D Engineer
- R&D Manager
- IP Professional
- Industry Leading Data Capabilities
- Powerful AI technology
- Patent DNA Extraction
Browse by: Latest US Patents, China's latest patents, Technical Efficacy Thesaurus, Application Domain, Technology Topic, Popular Technical Reports.
© 2024 PatSnap. All rights reserved.Legal|Privacy policy|Modern Slavery Act Transparency Statement|Sitemap|About US| Contact US: help@patsnap.com