Deep stack network-based electroencephalogram signal feature extraction and classification method
An EEG signal and stacking network technology, which is applied in the direction of instruments, character and pattern recognition, computer components, etc., can solve the problems of EEG signal recognition process analysis, consume a lot of manpower and material resources, and reduce the generalization ability of the model. The effect of reducing the gradient dispersion problem, improving the classification recognition rate, and facilitating parallel operations
- Summary
- Abstract
- Description
- Claims
- Application Information
AI Technical Summary
Problems solved by technology
Method used
Image
Examples
Embodiment Construction
[0041] The present invention will be further described below in conjunction with drawings and embodiments.
[0042] As shown in the figure, the EEG feature extraction and classification method based on the deep stack network provided by this embodiment includes the following steps:
[0043] (1) To collect EEG signal data, the EEG signal acquisition device adopts Emotiv EEG signal acquisition instrument. Emotiv contains a total of 16 electrodes, of which CMS and DRL are two reference electrodes, and the electrodes are placed according to the international 10-20 standard electrode placement method. The sampling frequency of the signal is 128Hz. After the collected EEG signal is amplified and filtered, it is transmitted to the computer through the wireless USB receiver. The experiment was carried out in a relatively quiet environment. At the beginning of the experiment (t=0s), the subject sat quietly on the chair and kept in a relaxed state; at t=2s, the subject began to imagine...
PUM
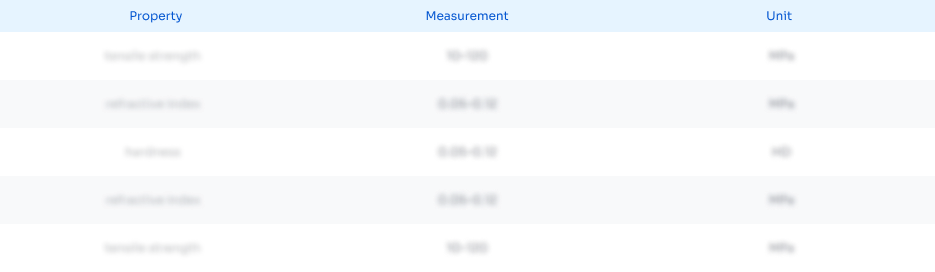
Abstract
Description
Claims
Application Information
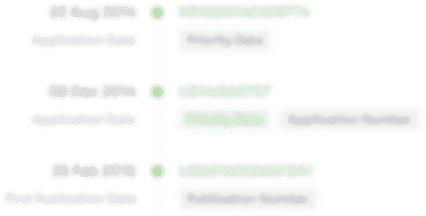
- R&D Engineer
- R&D Manager
- IP Professional
- Industry Leading Data Capabilities
- Powerful AI technology
- Patent DNA Extraction
Browse by: Latest US Patents, China's latest patents, Technical Efficacy Thesaurus, Application Domain, Technology Topic, Popular Technical Reports.
© 2024 PatSnap. All rights reserved.Legal|Privacy policy|Modern Slavery Act Transparency Statement|Sitemap|About US| Contact US: help@patsnap.com