Vehicle model recognition model construction method based on depth learning and vehicle model recognition method based on depth learning
A vehicle identification and deep learning technology, applied in the fields of computer vision and intelligent transportation, can solve the problems of lack of intra-class differences, classification of adjacent vehicle types, and insufficient discriminative power, so as to achieve rich semantic information, speed up network convergence, and improve vehicle models. The effect of classification accuracy
- Summary
- Abstract
- Description
- Claims
- Application Information
AI Technical Summary
Problems solved by technology
Method used
Image
Examples
Embodiment Construction
[0035] Preferred embodiments of the present invention are described below with reference to the accompanying drawings. Those skilled in the art should understand that these embodiments are only used to explain the technical principles of the present invention, and are not intended to limit the protection scope of the present invention.
[0036] The embodiment of the present invention includes a method for constructing a vehicle type identification model based on deep learning, and a vehicle type identification method based on the constructed vehicle type identification model.
[0037] A method for building a vehicle identification model based on deep learning in an embodiment of the present invention includes the following steps:
[0038] Step A1, select pictures containing vehicles, and mark the vehicle position for each vehicle picture, specifically draw the smallest rectangular frame containing the vehicle in each vehicle picture, record the coordinate information of the up...
PUM
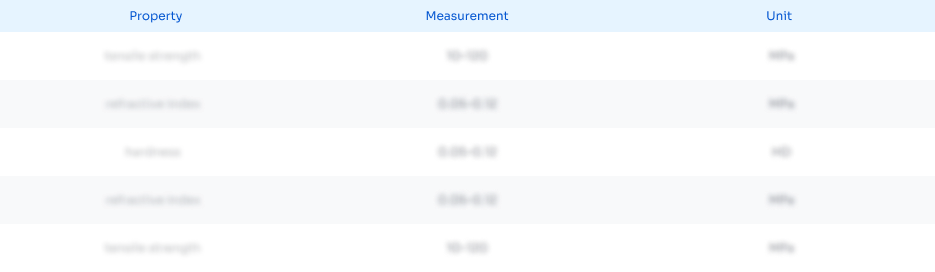
Abstract
Description
Claims
Application Information
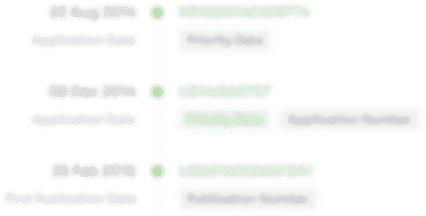
- R&D Engineer
- R&D Manager
- IP Professional
- Industry Leading Data Capabilities
- Powerful AI technology
- Patent DNA Extraction
Browse by: Latest US Patents, China's latest patents, Technical Efficacy Thesaurus, Application Domain, Technology Topic, Popular Technical Reports.
© 2024 PatSnap. All rights reserved.Legal|Privacy policy|Modern Slavery Act Transparency Statement|Sitemap|About US| Contact US: help@patsnap.com