Improved multidimensional scaling heterogeneous cost-sensitive decision tree building method
A cost-sensitive, construction method technology, applied in structured data retrieval, special data processing applications, instruments, etc., can solve the problem of low test cost, reduce the cost of misclassification, improve efficiency, and strengthen the classification ability.
- Summary
- Abstract
- Description
- Claims
- Application Information
AI Technical Summary
Problems solved by technology
Method used
Image
Examples
Embodiment Construction
[0031] Aiming at solving the problem of constructing a multi-dimensional scale decision tree process by considering the test cost, misclassification cost and waiting time cost influencing factors at the same time, the test cost is lower, the decision tree has better scalability, and the difference in cost The final decision tree generated by the unit mechanism problem better avoids the overfitting problem, combined with figure 1 The present invention has been described in detail, and its specific implementation steps are as follows:
[0032] Step 1: Suppose there are X samples in the training set, and the number of attributes is n, that is, n=(S 1 , S 2 ,…S n ), while splitting the attribute S i Corresponds to m classes L, where L r ∈(L 1 , L 2 ...,L m ), i ∈ (1, 2..., n), r ∈ (1, 2..., m). Users in related fields set the misclassification cost matrix C and attribute S i The test cost is cost i ,,wc(S i )—relative waiting time cost value, correction coefficient β, a...
PUM
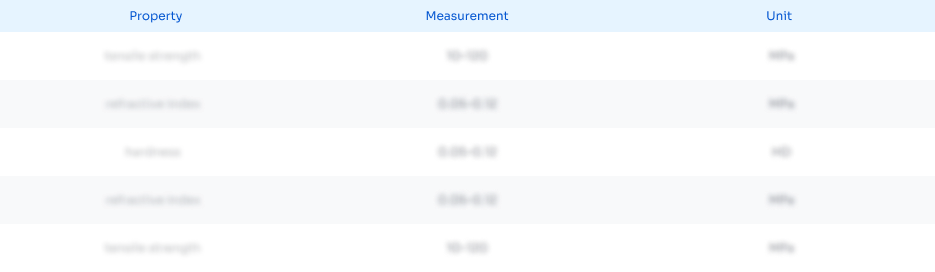
Abstract
Description
Claims
Application Information
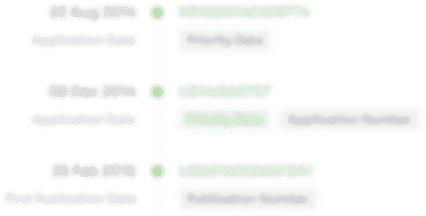
- Generate Ideas
- Intellectual Property
- Life Sciences
- Materials
- Tech Scout
- Unparalleled Data Quality
- Higher Quality Content
- 60% Fewer Hallucinations
Browse by: Latest US Patents, China's latest patents, Technical Efficacy Thesaurus, Application Domain, Technology Topic, Popular Technical Reports.
© 2025 PatSnap. All rights reserved.Legal|Privacy policy|Modern Slavery Act Transparency Statement|Sitemap|About US| Contact US: help@patsnap.com