Transformer fault classification and identification method based on vibration analysis method
A technology for transformer faults and identification methods, which is applied to instruments, measuring electrical variables, measuring devices, etc., and can solve problems such as inability to achieve nonlinear classification, adverse observation, and influence.
- Summary
- Abstract
- Description
- Claims
- Application Information
AI Technical Summary
Problems solved by technology
Method used
Image
Examples
Embodiment 1
[0060] Such as figure 1 The shown transformer fault classification and identification method based on the vibration analysis method includes the following steps:
[0061] S1: Select the corresponding transformer as the test object, and collect the vibration signals of the transformer in different states as sample data;
[0062] S2: Using the set empirical mode decomposition method in the Hilbert-Huang transform to calculate the sample data to obtain the eigenmode function;
[0063] S3: extracting the characteristic vector V in the intrinsic modulus function component;
[0064] S4: Use the principal component analysis method to reduce the dimensionality of the feature vector, and project the coordinates into the two-dimensional image;
[0065] S5: Classify the sample data using the K-nearest method;
[0066] S6: Using the distance formula to calculate the distance between the test sample and the original sample;
[0067] S7: performing pattern recognition;
[0068] S8: Out...
Embodiment 2
[0070] On the basis of the foregoing examples, specifically:
[0071] In step S2, the steps of calculating the sample data to obtain the intrinsic modulus function by using the set empirical mode decomposition method in the Hilbert-Huang transform are as follows:
[0072] Add M times of Gaussian white noise sequence n to the original signal x(t) i (t), (i=1, 2, ..., M), namely:
[0073] ;
[0074] to x i (t) Carry out EMD respectively to obtain the components and margins of each eigenmode function, namely:
[0075] ;
[0076] where h ij After adding Gaussian white noise for the ith time, for X i (t) the jth eigenmode function component obtained by decomposing; r in After adding Gaussian white noise for the ith time, for X i (t) remainder after decomposing; n is the number of decomposition levels;
[0077] Using the zero-mean principle of the Gaussian white noise spectrum, the eigenmode function components corresponding to the above steps are generally averaged to ...
PUM
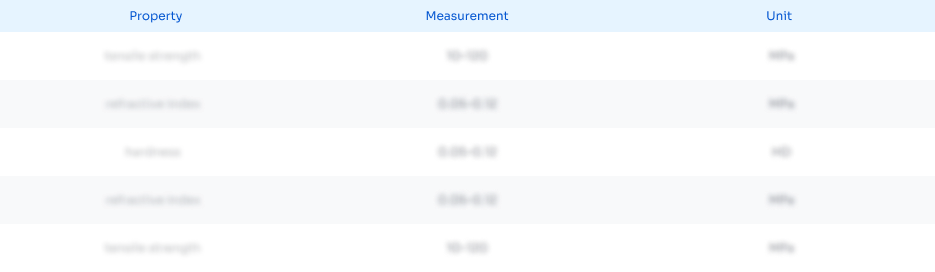
Abstract
Description
Claims
Application Information
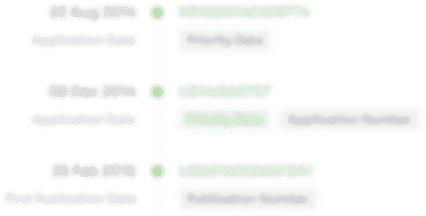
- R&D Engineer
- R&D Manager
- IP Professional
- Industry Leading Data Capabilities
- Powerful AI technology
- Patent DNA Extraction
Browse by: Latest US Patents, China's latest patents, Technical Efficacy Thesaurus, Application Domain, Technology Topic, Popular Technical Reports.
© 2024 PatSnap. All rights reserved.Legal|Privacy policy|Modern Slavery Act Transparency Statement|Sitemap|About US| Contact US: help@patsnap.com