Kernel sparse representation-based fast remote sensing target detection and recognition method
A technology of kernel sparse representation and target detection, applied in the field of remote sensing image analysis, can solve problems such as loss detection and recognition performance, capture similarity between features, and limit the scope of application, so as to reduce redundant calculation, speed up, and speed up image description. Effect
- Summary
- Abstract
- Description
- Claims
- Application Information
AI Technical Summary
Problems solved by technology
Method used
Image
Examples
Embodiment Construction
[0033] This specific embodiment discloses a fast remote sensing target detection and recognition method based on kernel sparse representation, such as figure 1 shown, including the following steps:
[0034] S1: Create four RGB feature channels RG(t), BY(t), I(t) and M(t), as shown in formulas (1)-(4);
[0035] RG(t)=R(t)-G(t) (1)
[0036] BY(t)=R(t)-G(t) (2)
[0037]
[0038] M(t)=|I(t)-I(t-τ)| (4)
[0039] Among them, r(t), g(t), b(t) respectively represent the RGB three channels of the image, and R(t), G(t) and B(t) are shown in formulas (5)-(7), I(t) represents the image at time t;
[0040]
[0041]
[0042]
[0043] S2: Calculate the four-phase Fourier transform of the four feature channels of a given image, extract the phase spectrum, and reconstruct the images of the four feature channels by inverse Fourier transform, thereby generating a saliency map.
[0044] S3: Binarize the saliency map obtained in step S2, and extract candidate regions of interest; ...
PUM
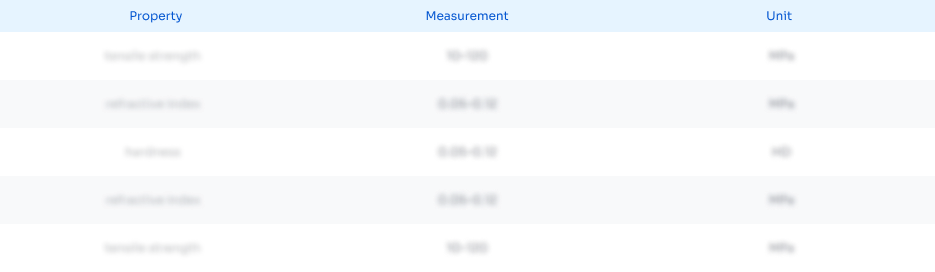
Abstract
Description
Claims
Application Information
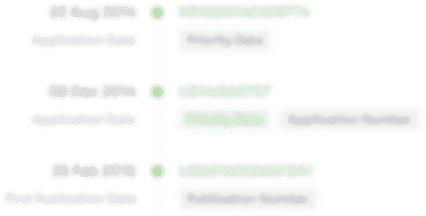
- R&D
- Intellectual Property
- Life Sciences
- Materials
- Tech Scout
- Unparalleled Data Quality
- Higher Quality Content
- 60% Fewer Hallucinations
Browse by: Latest US Patents, China's latest patents, Technical Efficacy Thesaurus, Application Domain, Technology Topic, Popular Technical Reports.
© 2025 PatSnap. All rights reserved.Legal|Privacy policy|Modern Slavery Act Transparency Statement|Sitemap|About US| Contact US: help@patsnap.com