Image understanding method based on depth residual error network and LSTM
An image understanding and residual technology, applied in the field of deep learning and image semantic understanding, can solve problems such as difficulty in implementation, low recognition rate, and poor generalization
- Summary
- Abstract
- Description
- Claims
- Application Information
AI Technical Summary
Problems solved by technology
Method used
Image
Examples
Embodiment
[0060] Such as figure 1 Shown is the method flowchart of the present invention, comprises the steps:
[0061] (1) Download training data sets: Download ImageNet and MS-COCO public image data sets from http: / / www.image-net.org and http: / / mscoco.org respectively. The ImageNet dataset is divided into a training image set and a test image set. The training image set contains 1,000 categories of pictures, 1,300 for each category, and the test image set contains 50,000 pictures; the MS-COCO dataset is divided into a training image set and a test image set. , the training image set contains 82,783 pictures, and the test image set contains 40,504 pictures. Correspondingly, each picture has 5 natural language sentences used to describe its content information.
[0062] (2), pretreatment:
[0063] For the ImageNet dataset: for each image, the image is scaled to a size of 256×256, and then 5 standard-size images with a size of 224×224 are intercepted from the top, middle, bottom, left,...
PUM
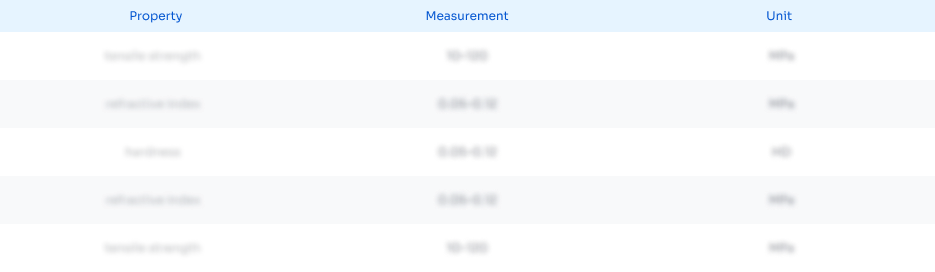
Abstract
Description
Claims
Application Information
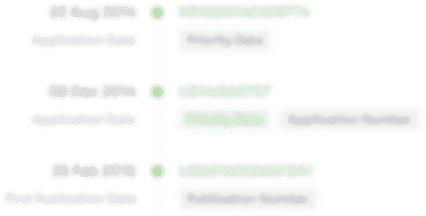
- R&D Engineer
- R&D Manager
- IP Professional
- Industry Leading Data Capabilities
- Powerful AI technology
- Patent DNA Extraction
Browse by: Latest US Patents, China's latest patents, Technical Efficacy Thesaurus, Application Domain, Technology Topic.
© 2024 PatSnap. All rights reserved.Legal|Privacy policy|Modern Slavery Act Transparency Statement|Sitemap