Deep learning super-resolution reconstruction method based on residual sub-images
A super-resolution reconstruction and deep learning technology, applied in the field of computer image processing, can solve problems such as blurring effect, reconstruction effect, nonlinear feature representation ability and limited image reconstruction ability, so as to improve reconstruction speed and quality , enhance the effect of visual effects
- Summary
- Abstract
- Description
- Claims
- Application Information
AI Technical Summary
Problems solved by technology
Method used
Image
Examples
Embodiment Construction
[0043] Such as Figure 1-8 As shown in one of them, the present invention discloses a deep learning super-resolution reconstruction method based on the residual sub-image, which cleverly combines the residual sub-image and the deep learning method based on the convolutional neural network, which can not only reconstruct high-resolution High-quality images or videos provide a good viewing experience for users, and can quickly reconstruct high-resolution images. The specific implementation process is as image 3 as shown,
[0044] It includes the following steps:
[0045] 1) Train the deep neural network model:
[0046] 1-1) If figure 1 As shown, the high-resolution image y is decomposed into s 2 subimage y sub ; where s is the super-resolution magnification of the image. The sub-image is valued every s pixels in the rows and columns of the high-resolution image. If the input image is in RGB space, it needs to be converted to YCbCr space first, and the algorithm is only ...
PUM
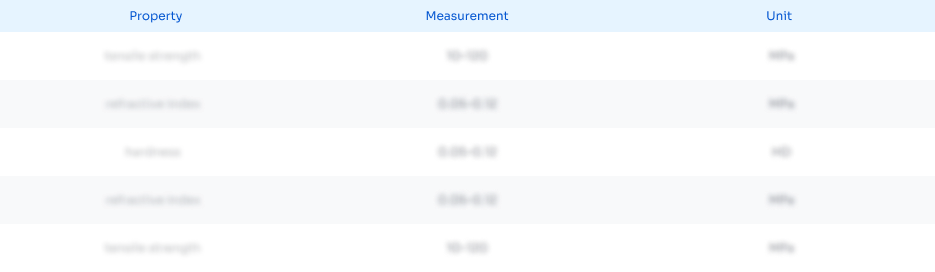
Abstract
Description
Claims
Application Information
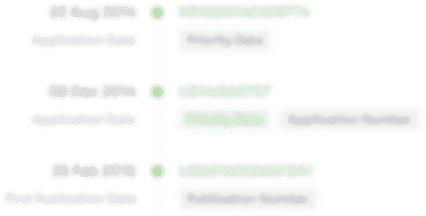
- R&D
- Intellectual Property
- Life Sciences
- Materials
- Tech Scout
- Unparalleled Data Quality
- Higher Quality Content
- 60% Fewer Hallucinations
Browse by: Latest US Patents, China's latest patents, Technical Efficacy Thesaurus, Application Domain, Technology Topic, Popular Technical Reports.
© 2025 PatSnap. All rights reserved.Legal|Privacy policy|Modern Slavery Act Transparency Statement|Sitemap|About US| Contact US: help@patsnap.com