Joint pre-stack elastic inversion parameter and deep network target inversion technology
A deep network, elastic impedance inversion technology, applied in the field of target inversion, can solve the problems of increasing cumulative error, result inaccuracy, and reducing accuracy, so as to improve the degree of representation, enhance the reliability and authenticity, and improve the The effect of accuracy
- Summary
- Abstract
- Description
- Claims
- Application Information
AI Technical Summary
Problems solved by technology
Method used
Image
Examples
Embodiment 1
[0037] refer to figure 1 , this embodiment discloses a joint pre-stack elastic inversion parameter and a deep network target inversion technology. The specific steps of the target inversion technology are as follows:
[0038] S1. Calculate the well data to obtain the pre-stack elastic impedance inversion parameters;
[0039] Specifically, the pre-stack elastic impedance inversion parameters include compressional wave velocity, shear wave velocity and density.
[0040] S2. Carry out an angle division on the side channel pre-stack seismic data, and obtain the pre-stack parameters of the division angle;
[0041] Specifically, the pre-stack parameters for dividing angles include large-angle data, medium-angle data, and small-angle data.
[0042] S3. Taking the well bypass data corresponding to each time point on the well bypass as input, and the well bypass target data at this time point as output constraints;
[0043] S4, establishing a training network;
[0044] In addition,...
Embodiment 2
[0049] refer to figure 2 , this embodiment discloses joint pre-stack elastic inversion parameters and deep network target inversion technology; this embodiment mainly describes the deep neural network algorithm except for the same steps as embodiment 1;
[0050] In this embodiment, the deep neural network algorithm (DNN) is used to establish the relationship between the label data set and the training data set to form a prediction network, which uses neural network technology, which is also called a perceptron, and has an input layer, The output layer and a hidden layer. The input feature vector reaches the output layer through the hidden layer transformation, and the classification result is obtained in the output layer. DNN can be understood as a neural network with many hidden layers;
[0051] It needs to be further explained that the deep neural network algorithm can be divided according to the position of different layers, and its internal neural network layers can be di...
PUM
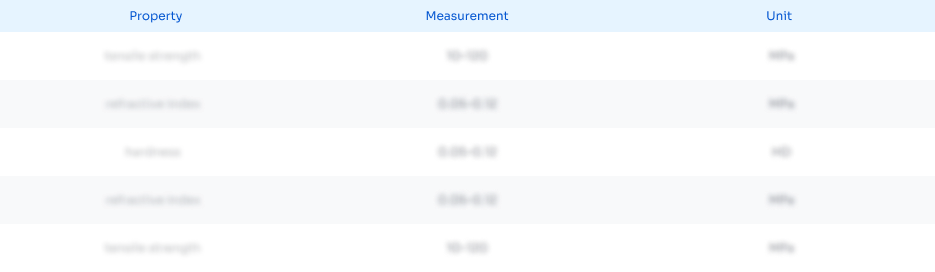
Abstract
Description
Claims
Application Information
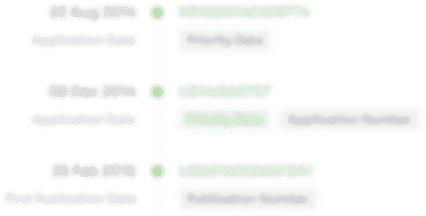
- R&D
- Intellectual Property
- Life Sciences
- Materials
- Tech Scout
- Unparalleled Data Quality
- Higher Quality Content
- 60% Fewer Hallucinations
Browse by: Latest US Patents, China's latest patents, Technical Efficacy Thesaurus, Application Domain, Technology Topic, Popular Technical Reports.
© 2025 PatSnap. All rights reserved.Legal|Privacy policy|Modern Slavery Act Transparency Statement|Sitemap|About US| Contact US: help@patsnap.com