Matrix decomposition cross-model Hash retrieval method on basis of cooperative training
A matrix decomposition and co-training technology, applied in the field of image processing, can solve the problems of low discriminative hash coding, affecting retrieval accuracy, and inability to effectively maintain the similarity between modalities and the similarity within modalities at the same time. The effect of mutual retrieval performance and improving mutual retrieval accuracy
- Summary
- Abstract
- Description
- Claims
- Application Information
AI Technical Summary
Problems solved by technology
Method used
Image
Examples
Embodiment 1
[0037]In the era of big data, the acquisition and processing of information is very important, and retrieval technology is a key step, especially in the context of the emergence of a large number of various modal data, how to effectively retrieve is also the key to information utilization. The existing cross-modal hash retrieval methods cannot effectively maintain the inter-modal and intra-modal similarity at the same time when the class label information is not easy to obtain in practice, and the retrieval accuracy is also affected. In response to this problem, the present invention has carried out innovative research, and proposed a matrix decomposition cross-modal hash retrieval method based on collaborative training, see figure 1 , the entire hash retrieval process includes the following steps:
[0038] (1) Obtain the original data, the original data set includes the training data set and the test data set, normalize the training data of the original data set, and obtain t...
Embodiment 2
[0060] The matrix decomposition cross-modal hash retrieval method based on collaborative training is the same as embodiment 1, the neighbor graph of the construction training data described in step (3), obtains the neighbor relationship of the training data, and proceeds as follows:
[0061] (3a) Each row of the normalized image training data matrix is regarded as a vector as an image data, and the Euclidean distance d between every two vectors is obtained;
[0062] (3b) Sort the Euclidean distance d, and for each image data, take out the Euclidean distances of its k nearest neighbors, and save it into a symmetrical adjacency matrix W 1 , the value range of k is [10, 50]. When the value of k is large, the accuracy will be improved but the amount of calculation will be increased. The value of k is also related to the amount of data in the retrieved system. In this example, the number of neighbors k is 10;
[0063] (3c) Calculate the image data adjacency matrix W 1 The Lapla...
Embodiment 3
[0068] The matrix decomposition cross-modal hash retrieval method based on collaborative training is the same as embodiment 1-2, wherein the process of obtaining the objective function in step (4) includes:
[0069] (4a) Image training data X respectively (1) and text training data X (2) Perform matrix decomposition, construct matrix decomposition reconstruction error term Where||·|| F Represents the F-norm of the matrix, U 1 , U 2 are the base matrices of image data and text data respectively, V is the same coefficient matrix of paired image and text data under the base matrix, α is the balance parameter between the two modalities, take α=0.5, the two modal The data contribute equally to the objective function.
[0070] (4b) Since the training data X t The hash coding of is obtained by quantizing the low-dimensional representation coefficient V, so the linear projection reconstruction error term is constructed Get the linear projection matrix W of the training data ...
PUM
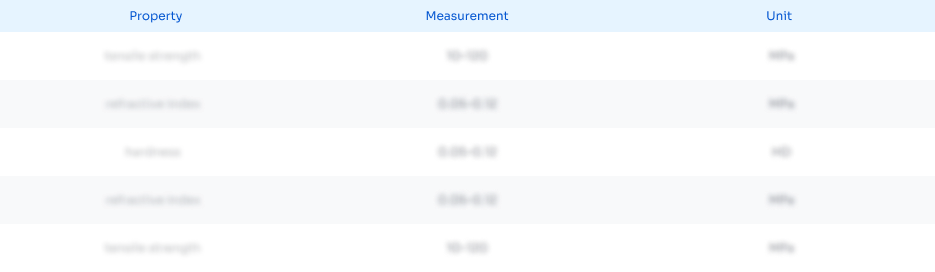
Abstract
Description
Claims
Application Information
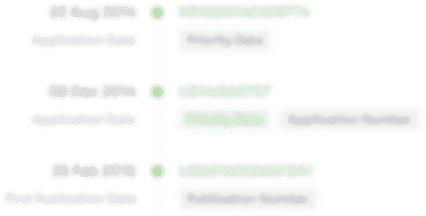
- R&D Engineer
- R&D Manager
- IP Professional
- Industry Leading Data Capabilities
- Powerful AI technology
- Patent DNA Extraction
Browse by: Latest US Patents, China's latest patents, Technical Efficacy Thesaurus, Application Domain, Technology Topic, Popular Technical Reports.
© 2024 PatSnap. All rights reserved.Legal|Privacy policy|Modern Slavery Act Transparency Statement|Sitemap|About US| Contact US: help@patsnap.com