Medical image retrieval method based on deep learning and Radon conversion
A medical image and deep learning technology, applied in the field of computer vision and image retrieval, can solve problems such as high complexity, and achieve the effect of reducing the dimension of feature vectors, overcoming the semantic gap, reducing computational complexity and time consumption
- Summary
- Abstract
- Description
- Claims
- Application Information
AI Technical Summary
Problems solved by technology
Method used
Image
Examples
Embodiment Construction
[0052] In order to make the purpose, technical solution and related advantages of the present invention clearer, the present invention will be further described in detail below in conjunction with the accompanying drawings and specific embodiments.
[0053] The present invention proposes a medical image retrieval method based on deep learning and Radon transformation. The method uses the BING target suggestion algorithm to block the input image, then inputs the constructed deep convolutional network, and introduces partial mean value Pooling into the network at the same time. Extract distinguishing features. In the process of similarity measurement, the product quantization algorithm is introduced to effectively reduce the computational complexity and obtain the "rough" retrieval results. On this basis, the "rough" retrieval results are more accurate using the Radon transform method. Retrieve, and finally get the Top 10 images most similar to the query image. The key steps of ...
PUM
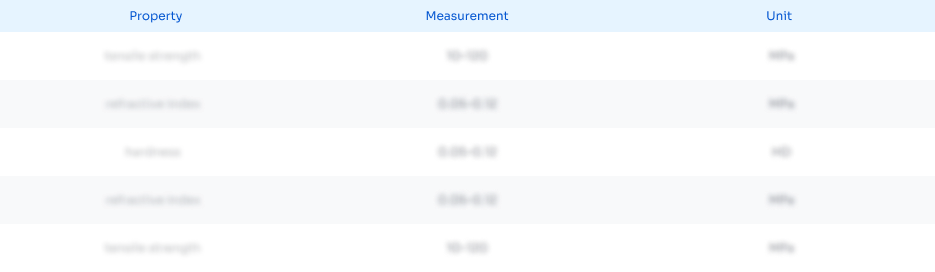
Abstract
Description
Claims
Application Information
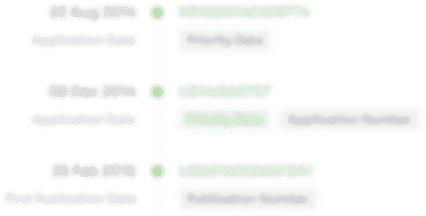
- R&D Engineer
- R&D Manager
- IP Professional
- Industry Leading Data Capabilities
- Powerful AI technology
- Patent DNA Extraction
Browse by: Latest US Patents, China's latest patents, Technical Efficacy Thesaurus, Application Domain, Technology Topic, Popular Technical Reports.
© 2024 PatSnap. All rights reserved.Legal|Privacy policy|Modern Slavery Act Transparency Statement|Sitemap|About US| Contact US: help@patsnap.com