A Spectral Tensor Dimensionality Reduction and Classification Method Based on Tucker Decomposition
A classification method and spectrum technology, applied in the field of remote sensing image processing, to achieve the effect of improving classification accuracy and stable results
- Summary
- Abstract
- Description
- Claims
- Application Information
AI Technical Summary
Problems solved by technology
Method used
Image
Examples
Embodiment 1
[0042] This embodiment provides a spectral tensor dimensionality reduction method based on Tucker decomposition, which uses randomly selected pixel spectra as intra-class factors, and constructs intra-class factors, class and pixel spectrum bands as a model respectively. A rank 3 tensor, which is subjected to dimensionality reduction based on low-rank tensor decomposition;
[0043] Step 1. In this embodiment, the pixel spectrum in the hyperspectral image of Washington DC Mall of HYDICE is selected, and the original image size is 1280×307. There are a total of 210 bands from 0.4 to 2.4 μm in the visible to infrared spectrum. Due to the opacity of the atmosphere, the bands in the 0.9 to 1.4um region are discarded, and the remaining 191 bands are used as bands in the pixel spectrum. Such as figure 2 As shown, the hyperspectral image includes 7 types of samples, namely: roof (Roof), street (street), lawn (Grass), tree (Tree), path (Path), water body (Water) and shadow (Shadow); ...
Embodiment 2
[0048] In this embodiment, the pixel spectrum of the hyperspectral image selected in Embodiment 1 is used as the training set, and any unclassified pixel spectrum in Washington DC Mall of HYDICE is input as the test pixel spectrum d.
[0049] 其中,d为(0.4012,0.3909,0.3885,0.4026,0.4004,0.3967,0.3778,0.3441,0.3792,0.4121,0.4219,0.4552,0.4969,0.5005,0.501,0.4889,0.4709,0.4665,0.4226,0.4888,0.3852,0.3612,0.3712 ,0.3781,0.3739,0.3624,0.3492,0.3297,0.3159,0.3148,0.3036,0.3096,0.2995,0.2951,0.2817,0.268,0.2558,0.2423,0.2392,0.231,0.2339,0.2303,0.2225,0.2131,0.2022,0.2024,0.206,0.1995 ,0.2006,0.2028,0.1943,0.175,0.1735,0.1809,0.17,0.1621,0.1744,0.1789,0.145,0.1487,0.1724,0.1647,0.1514,0.1302,0.1329,0.4095,0.4073,0.4038,0.3902,0.3184,0.2357,0.1458,0.116 ,0.1377,0.2284,0.2996,0.3431,0.3453,0.3418,0.3389,0.3138,0.2863,0.2684,0.2084,0.0903,0.0497,0.0664,0.132,0.1774,0.186,0.1915,0.2055,0.2131,0.2077,0.1824,0.1871,0.1831,0.1501 ,0.1049,0.0579,0.0128,0.0034,0.0062,0.0155,0.0193,0.0213,0.0441...
PUM
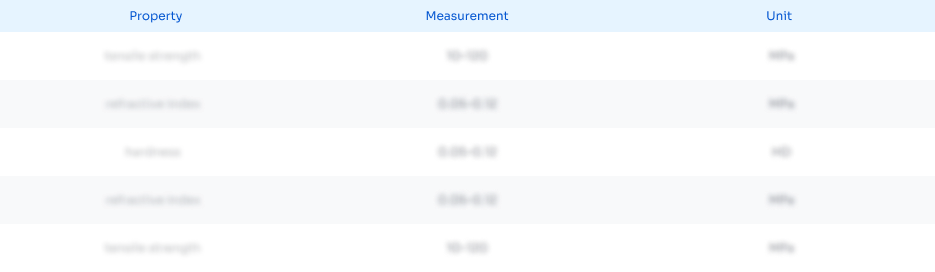
Abstract
Description
Claims
Application Information
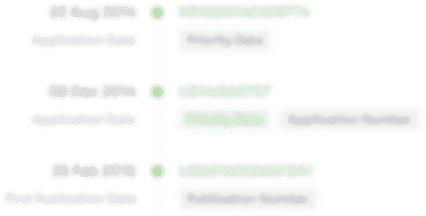
- R&D Engineer
- R&D Manager
- IP Professional
- Industry Leading Data Capabilities
- Powerful AI technology
- Patent DNA Extraction
Browse by: Latest US Patents, China's latest patents, Technical Efficacy Thesaurus, Application Domain, Technology Topic, Popular Technical Reports.
© 2024 PatSnap. All rights reserved.Legal|Privacy policy|Modern Slavery Act Transparency Statement|Sitemap|About US| Contact US: help@patsnap.com