Method for learning driving style based on self-coded regularization network
A driving style and network learning technology, applied in the field of driving recognition, can solve problems such as difficult to describe the driving style of unknown drivers
- Summary
- Abstract
- Description
- Claims
- Application Information
AI Technical Summary
Problems solved by technology
Method used
Image
Examples
Embodiment Construction
[0036] It should be noted that, in the case of no conflict, the embodiments in the present application and the features in the embodiments can be combined with each other. The present invention will be further described in detail below in conjunction with the drawings and specific embodiments.
[0037] figure 1 It is a system flowchart of a method for learning driving style based on self-encoding regularization network of the present invention. Mainly including GPS data conversion, self-encoder regularization network, objective function and approximation, run-length encoding framework, driver population estimation.
[0038] Wherein, the described GPS data conversion defines the itinerary (i.e. the GPS track) by a tuple sequence (u, v, t) of varying length, where (u, v) represents the geographic location, and t represents the time, from the original GPS data To construct the neural network input, a trip is first divided into fixed length L s and press L s A window of / 2 disp...
PUM
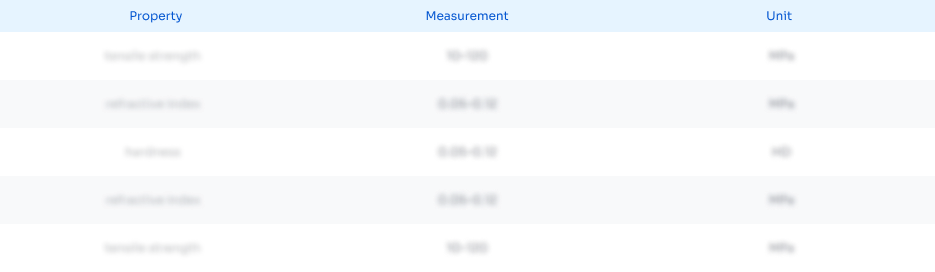
Abstract
Description
Claims
Application Information
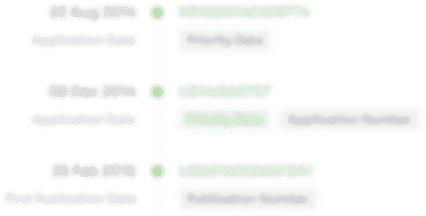
- Generate Ideas
- Intellectual Property
- Life Sciences
- Materials
- Tech Scout
- Unparalleled Data Quality
- Higher Quality Content
- 60% Fewer Hallucinations
Browse by: Latest US Patents, China's latest patents, Technical Efficacy Thesaurus, Application Domain, Technology Topic, Popular Technical Reports.
© 2025 PatSnap. All rights reserved.Legal|Privacy policy|Modern Slavery Act Transparency Statement|Sitemap|About US| Contact US: help@patsnap.com