Gaming-based link predication method and gaming-based link predication system in exchangeable graph
A graph representation and data concentration technology, applied in the field of machine learning, can solve the problems of model training efficiency and accuracy impact, low degree of parallelization, no observation, etc., achieve high accuracy, improve training speed, and improve training speed Effect
- Summary
- Abstract
- Description
- Claims
- Application Information
AI Technical Summary
Problems solved by technology
Method used
Image
Examples
Embodiment 1
[0039] A game-based link prediction method in a commutative graph, using the following steps:
[0040] (1) Obtain the data set, and represent the elements in the data set through a graph. The graph is composed of a node set and an edge set; the nodes represent the entities to be predicted, and the edges represent the relationship between the entities to be predicted. The nodes of the data set and edges satisfy the requirements of commutative graphs;
[0041] (2) using a game-based network evolution model to filter edges that do not meet the set requirements in the graph, and use the filtered graph as a training set;
[0042] (3) Using the training set to iteratively train the probability graph model to obtain a probability graph model with optimal model parameters;
[0043] (4) Use the probabilistic graphical model in (3) with optimal model parameters for link prediction.
[0044] Based on the game-based network evolution model NFG, the utility function of each network evolu...
Embodiment 2
[0123] Embodiment 2: a game-based link prediction system in an exchangeable graph: it is characterized in that it includes:
[0124] The obtaining module is used to obtain the data set, and the elements in the data set are represented by a graph, and the graph is composed of a node set and an edge set; the nodes represent the entities to be predicted, and the edges represent the relationship between the entities to be predicted, and the data set The nodes and edges of meet the requirements of commutative graphs;
[0125] A filtering module, configured to use a game-based network evolution model to filter edges that do not meet the set requirements in the graph, and use the filtered graph as a training set;
[0126] The training module is used to iteratively train the probability graph model using the training set to obtain the probability graph model with optimal model parameters;
[0127] A prediction module, configured to use the probabilistic graphical model with...
Embodiment 3
[0130] Embodiment 3: The present invention acquires data sets published on the Internet, including Highschool, NIPS, Protein, etc., as shown in Table 1.
[0131] Among them, Highschool is a network among students, which is used to describe the mutual understanding between students. Through this data set, it is possible to predict which students will know each other and which students will be more likely to form a small group together.
[0132] NIPS includes authors and papers published in NIPS 1-17 conferences. Among them, we selected a subset including 234 authors and their co-authored paper relationship for example analysis. Through this data set, our prediction method can be applied to judge whether it is easy to form cooperation between two scholars, so as to better cluster the scholar groups.
[0133] Protein data describes the link relationship between proteins. On this data set, our method can be used to predict the relationship between unknown proteins, there...
PUM
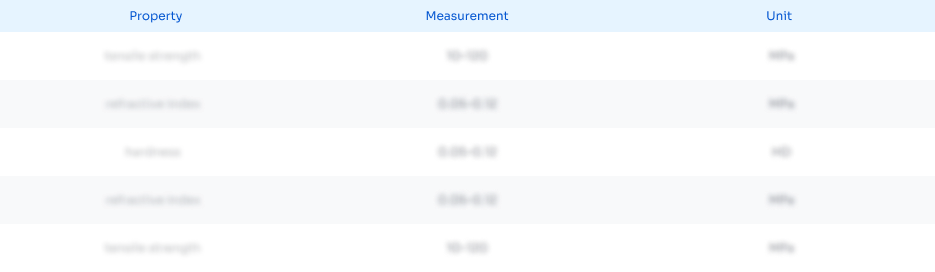
Abstract
Description
Claims
Application Information
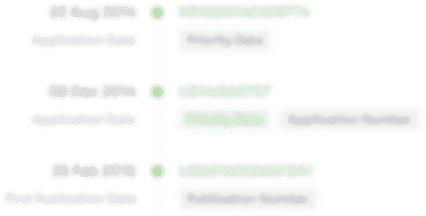
- R&D
- Intellectual Property
- Life Sciences
- Materials
- Tech Scout
- Unparalleled Data Quality
- Higher Quality Content
- 60% Fewer Hallucinations
Browse by: Latest US Patents, China's latest patents, Technical Efficacy Thesaurus, Application Domain, Technology Topic, Popular Technical Reports.
© 2025 PatSnap. All rights reserved.Legal|Privacy policy|Modern Slavery Act Transparency Statement|Sitemap|About US| Contact US: help@patsnap.com