Monitoring video multi-granularity marking method based on generalized multi-labeling learning
A multi-label learning and monitoring video technology, applied in the field of computer vision, can solve the problems of difficult unified definition, single labeling, complex and changeable real scenes, etc., to achieve the effect of enhancing robustness and reducing false alarm rate and missed detection rate.
- Summary
- Abstract
- Description
- Claims
- Application Information
AI Technical Summary
Problems solved by technology
Method used
Image
Examples
Embodiment Construction
[0031] The implementation of the present invention will be described in detail below with reference to the drawings and examples, so as to fully understand and implement the implementation process of how to use technical means to solve technical problems and achieve technical effects in the present invention.
[0032] The invention discloses a monitoring video multi-granularity labeling method based on generalized multi-label learning, which is characterized in that, with the background of public security video monitoring content analysis, the research work is carried out from the theory and method of multi-level acquisition of video features and multi-granularity representation . First, based on the theory of multi-label learning and deep learning, analyze and extract the features of different levels of objects in the video, construct a generalized multi-label classification algorithm, and identify multiple targets of different categories in the surveillance video; secondly, b...
PUM
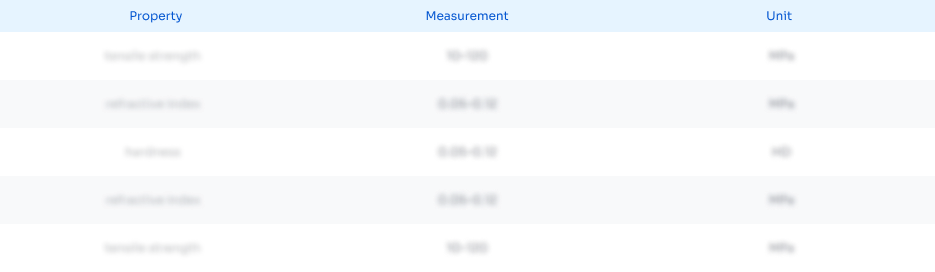
Abstract
Description
Claims
Application Information
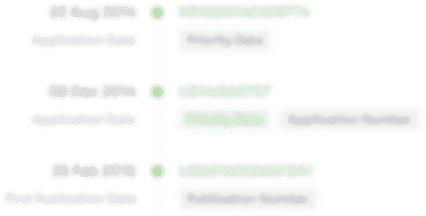
- R&D Engineer
- R&D Manager
- IP Professional
- Industry Leading Data Capabilities
- Powerful AI technology
- Patent DNA Extraction
Browse by: Latest US Patents, China's latest patents, Technical Efficacy Thesaurus, Application Domain, Technology Topic, Popular Technical Reports.
© 2024 PatSnap. All rights reserved.Legal|Privacy policy|Modern Slavery Act Transparency Statement|Sitemap|About US| Contact US: help@patsnap.com