Prior knowledge fault diagnosis method based on Tennessee Eastman process
A priori knowledge and fault diagnosis technology, applied in character and pattern recognition, instruments, computer components, etc., can solve problems such as difficult to obtain decision functions, achieve good fault detection effect, improve accuracy, and improve verification standards
- Summary
- Abstract
- Description
- Claims
- Application Information
AI Technical Summary
Problems solved by technology
Method used
Image
Examples
Embodiment Construction
[0052] The present invention will be further elaborated below in conjunction with the accompanying drawings of the description.
[0053] The present invention is based on the prior knowledge fault diagnosis method of Tennessee Eastman process and comprises the following steps:
[0054] 1) Collection of offline historical data X of Tennessee Eastman process X=[x 1 ,x 2 ,...,x l ,x l+1 ,...,x n ]∈R m×n , where x i (i=1,2,...,l) is the data that has been marked by expert prior knowledge, x i (i=l+1,l+2,...,n) is unlabeled data, l is the number of marked fault state categories, n is the total number of historical data fault state categories; initialization matrix Y∈R n×c , where c represents the fault state category, R m×n , R n×c Both represent the size of the data;
[0055] 2) Select the adjustment parameter matrix U∈R n×n and k in the KNN algorithm; among them, U∈R n×n Represents a diagonal matrix, k is the number of neighbor samples; (KNN, that is, the K nearest ne...
PUM
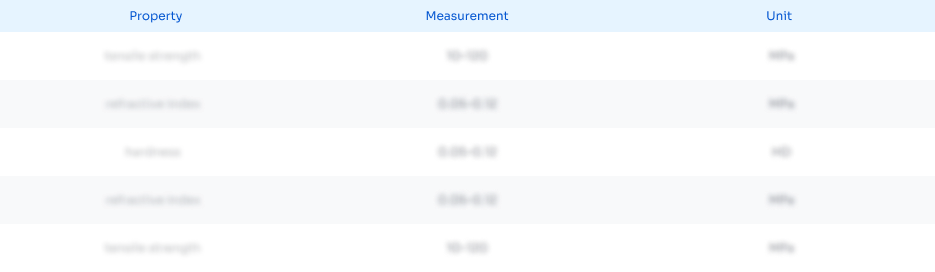
Abstract
Description
Claims
Application Information
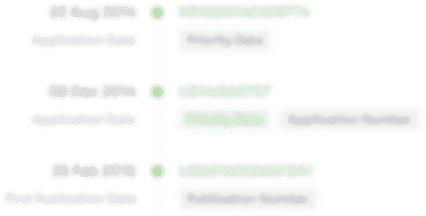
- R&D Engineer
- R&D Manager
- IP Professional
- Industry Leading Data Capabilities
- Powerful AI technology
- Patent DNA Extraction
Browse by: Latest US Patents, China's latest patents, Technical Efficacy Thesaurus, Application Domain, Technology Topic, Popular Technical Reports.
© 2024 PatSnap. All rights reserved.Legal|Privacy policy|Modern Slavery Act Transparency Statement|Sitemap|About US| Contact US: help@patsnap.com