Depth feature representation method based on multiple stacked auto-encoding
A technology of stacked self-encoding and deep features, which is applied in the field of deep feature representation based on multiple stacked self-encodings, which can solve the problem that the deep architecture can only extract single-layer structures.
- Summary
- Abstract
- Description
- Claims
- Application Information
AI Technical Summary
Problems solved by technology
Method used
Image
Examples
Embodiment Construction
[0038] First, explain the basic principles of stacked autoencoders. An autoencoder requires an input x=R d and the mapping h ∈ R of the input to the first latent representation d′ , using a deterministic function h=f θ =σ(W x +b), parameter θ={W,b}. Then use this way to reset the input by reverse mapping: y=f θ′ (h)=σ(W'h+b'), θ'={W', b'}. The two parameters are usually W'=W T is restricted in the form of encoding the input and decoding the latent representation y i use the same weights. The parameters will pass through the training set D n ={(x 0 , t 0 ),...(x n , t n )} to minimize an appropriate value function to be optimized.
[0039] First, build multiple multi-level autoencoders. This process is completely unsupervised, imitating the cognitive ability of the human brain, and realizing the process from rough to fine by combining features at different levels. Frameworks combine multiple autocoders, each of which has a different structure. A network with few...
PUM
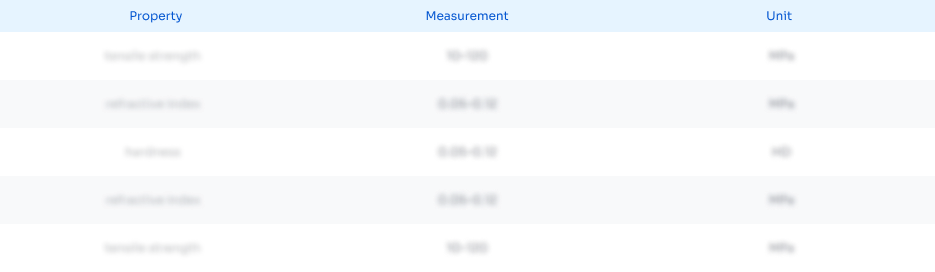
Abstract
Description
Claims
Application Information
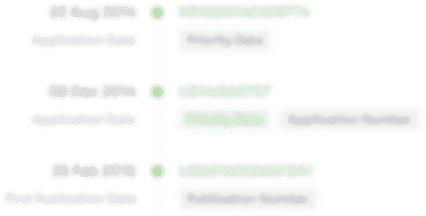
- R&D Engineer
- R&D Manager
- IP Professional
- Industry Leading Data Capabilities
- Powerful AI technology
- Patent DNA Extraction
Browse by: Latest US Patents, China's latest patents, Technical Efficacy Thesaurus, Application Domain, Technology Topic, Popular Technical Reports.
© 2024 PatSnap. All rights reserved.Legal|Privacy policy|Modern Slavery Act Transparency Statement|Sitemap|About US| Contact US: help@patsnap.com