End-to-end chest CT image segmentation method based on fully convolutional neural network
A convolutional neural network and CT image technology, applied in the field of medical image processing, can solve the problems of deformation model sensitivity, segmentation result error, and Snake model without standardized segmentation process, so as to achieve the effect of simplifying the process
- Summary
- Abstract
- Description
- Claims
- Application Information
AI Technical Summary
Problems solved by technology
Method used
Image
Examples
Embodiment Construction
[0042] The present invention is described in further detail now in conjunction with accompanying drawing. The drawings described here are used to provide a further understanding of the present invention and constitute a part of the application. The schematic embodiments of the present invention and their illustrations are only used to explain the present invention, and do not constitute improper limitations to the present invention.
[0043] Such as figure 1 Shown, the present invention comprises the following steps:
[0044] Step 1: Clinically scan k groups of chest CT images, and separate each group of CT images according to each slice as a training sample;
[0045] In the process of obtaining chest CT images, CT scanning is a spiral scan. The scanning process is generally that when the X-ray light source and detector rotate around the patient, the patient's bed moves slowly along the direction of the rotation axis. . Modern CT uses two-dimensional multi-row detectors to ...
PUM
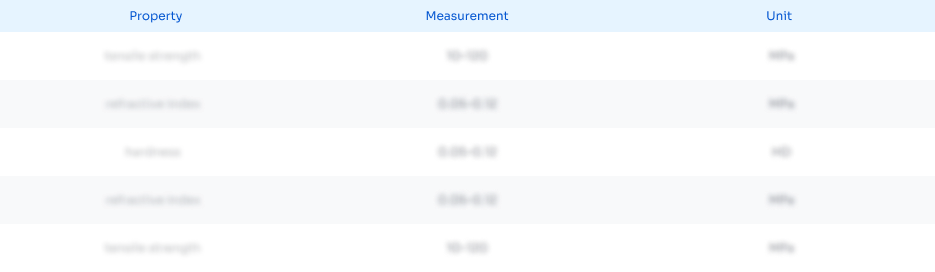
Abstract
Description
Claims
Application Information
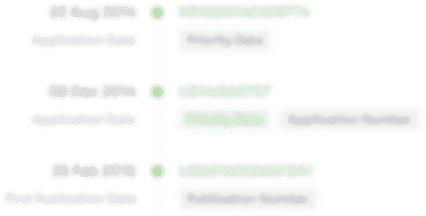
- R&D
- Intellectual Property
- Life Sciences
- Materials
- Tech Scout
- Unparalleled Data Quality
- Higher Quality Content
- 60% Fewer Hallucinations
Browse by: Latest US Patents, China's latest patents, Technical Efficacy Thesaurus, Application Domain, Technology Topic, Popular Technical Reports.
© 2025 PatSnap. All rights reserved.Legal|Privacy policy|Modern Slavery Act Transparency Statement|Sitemap|About US| Contact US: help@patsnap.com