Characteristic selection method based on sample characteristic distribution confusion degree
A feature selection method and sample feature technology, applied in the field of dimensionality reduction, can solve problems such as poor generalization ability, high selection efficiency, and high computational complexity, and achieve the effect of fast calculation speed and strong recognition ability
- Summary
- Abstract
- Description
- Claims
- Application Information
AI Technical Summary
Problems solved by technology
Method used
Image
Examples
Embodiment Construction
[0047] In order to make the purpose, technical solutions and advantages of the present invention clearer, the technical solutions of the present invention will be clearly and completely described below in conjunction with the accompanying drawings in the embodiments of the present invention. Obviously, the described embodiments are part of the implementation of the present invention. example, not all examples. Based on the embodiments of the present invention, all other embodiments obtained by persons of ordinary skill in the art without making creative efforts belong to the protection scope of the present invention.
[0048] The present invention designs a staged mixed feature selection method to effectively combine the Filter and Wrapper feature selection algorithms, and then complete the feature selection process in stages, that is, use the Filter method to quickly evaluate the features and then complete the specific classifier. The feature selection; specifically includes ...
PUM
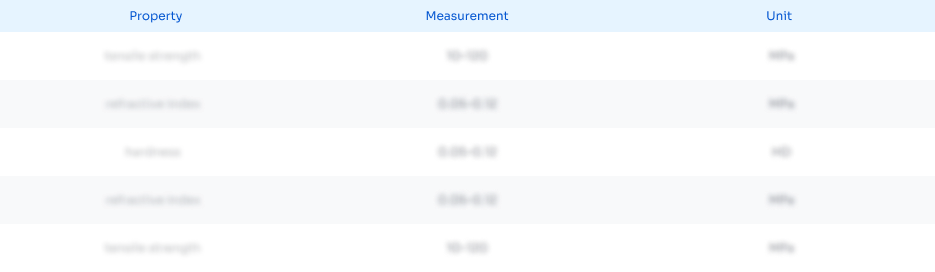
Abstract
Description
Claims
Application Information
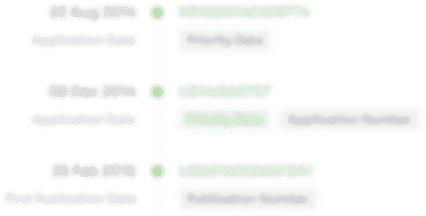
- R&D Engineer
- R&D Manager
- IP Professional
- Industry Leading Data Capabilities
- Powerful AI technology
- Patent DNA Extraction
Browse by: Latest US Patents, China's latest patents, Technical Efficacy Thesaurus, Application Domain, Technology Topic.
© 2024 PatSnap. All rights reserved.Legal|Privacy policy|Modern Slavery Act Transparency Statement|Sitemap