Generative adversarial network-based multi-pose face generation method
A face generation and multi-pose technology, applied in the field of deep learning, can solve problems such as the lack of multi-pose face database and the difficulty of multi-pose face recognition, and achieve the effect of improving the lack of large-scale data
- Summary
- Abstract
- Description
- Claims
- Application Information
AI Technical Summary
Problems solved by technology
Method used
Image
Examples
Embodiment
[0056] The implementation process of this embodiment is as follows:
[0057] 1) Collect multi-pose face images, organize and classify them according to the angle and pose information, and mark and encode them as the pose control parameter y;
[0058] Using the existing Multi_Pie database, which consists of 4 sessions, contains a total of 337 people in 15 poses and more than 750,000 pictures under 20 lighting conditions (although the database has a large number of face pictures, the number of people is relatively small, and To a large extent, it is the difference in illumination, not just the difference in posture), in this embodiment, only about 56,000 pictures under 7 postures of the first 200 people in the first session are used for training. Perform data preprocessing on the collected multi-pose face images. Data preprocessing includes operations such as mean subtraction (including mean subtraction in the image sense and mean subtraction based on the position of each pixel)...
PUM
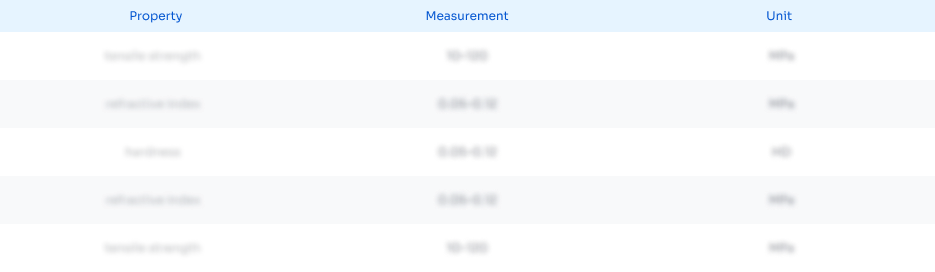
Abstract
Description
Claims
Application Information
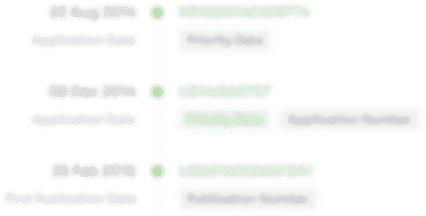
- R&D Engineer
- R&D Manager
- IP Professional
- Industry Leading Data Capabilities
- Powerful AI technology
- Patent DNA Extraction
Browse by: Latest US Patents, China's latest patents, Technical Efficacy Thesaurus, Application Domain, Technology Topic, Popular Technical Reports.
© 2024 PatSnap. All rights reserved.Legal|Privacy policy|Modern Slavery Act Transparency Statement|Sitemap|About US| Contact US: help@patsnap.com