Voice production method based on deep convolutional generative adversarial network
A deep convolution and network technology, applied in speech analysis, speech recognition, instruments, etc., can solve problems such as lack of change, monotonous patterns, discriminators cannot correctly distinguish generated data and real data, etc., to achieve easy understanding and acceptance Effect
- Summary
- Abstract
- Description
- Claims
- Application Information
AI Technical Summary
Problems solved by technology
Method used
Image
Examples
Embodiment
[0107] A speech generation method based on deep convolution generation confrontation network, the flow chart of its main method is as follows figure 1 As shown, it specifically includes the following steps:
[0108] (1) Collect speech signal samples: randomly collect 1000 speech signals with the same content ("Hello") as speech training samples and real speech samples respectively;
[0109] (2) Preprocessing of speech signals: Preprocessing is performed on the 1000 ("Hello") speech signals collected in step 1. First, use Audacity software to edit 1000 ("Hello") voice signals, and filter out the voice parts and non-voice signal parts in the original collected waveforms that are beyond the editing range of the software; secondly, perform voice filtering processing: through the filtering algorithm Calculate the error signal, correlation coefficient, and voice difference vector at n times, calculate the weight coefficient vector at each time by an iterative method, adjust the wei...
PUM
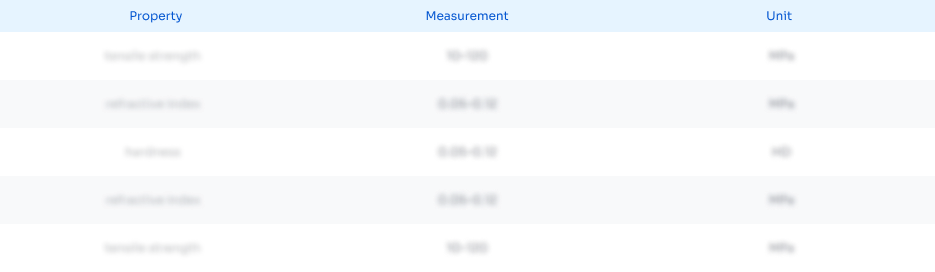
Abstract
Description
Claims
Application Information
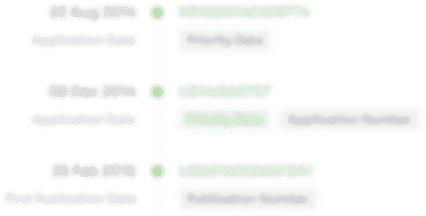
- R&D Engineer
- R&D Manager
- IP Professional
- Industry Leading Data Capabilities
- Powerful AI technology
- Patent DNA Extraction
Browse by: Latest US Patents, China's latest patents, Technical Efficacy Thesaurus, Application Domain, Technology Topic, Popular Technical Reports.
© 2024 PatSnap. All rights reserved.Legal|Privacy policy|Modern Slavery Act Transparency Statement|Sitemap|About US| Contact US: help@patsnap.com