Vehicle type recognition method based on CNN multiple-features combining and multi-core sparse representation
A sparse representation and vehicle recognition technology, applied in the field of vehicle recognition, which can solve the problems of irregular data, inability to effectively integrate heterogeneous data, and uneven samples.
- Summary
- Abstract
- Description
- Claims
- Application Information
AI Technical Summary
Problems solved by technology
Method used
Image
Examples
Embodiment Construction
[0056] The present invention is described in further detail now in conjunction with accompanying drawing.
[0057] Such as figure 1 The car model recognition method based on CNN multi-feature combination and multi-core sparse representation is shown, using the CNN network to extract the global and local features of the vehicle, and introducing it into the car model recognition based on sparse representation through multi-core weighted joint, so that the shallow and deep network The feature advantages are more fully and reasonably utilized, and multi-core learning makes the performance of different kernel functions more prominent, which can greatly improve the accuracy and robustness of vehicle identification. The specific steps are as follows.
[0058] Step 1: Vehicle Image Acquisition and Preprocessing
[0059] Using intelligent traffic cameras to capture images of four types of vehicles including large passenger cars, trucks, vans and cars in complex scenes, a total of 3,00...
PUM
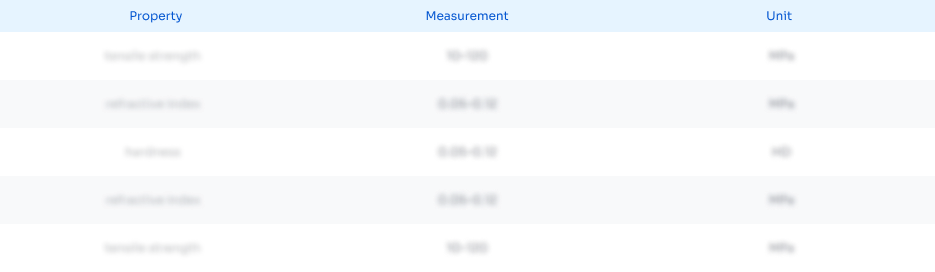
Abstract
Description
Claims
Application Information
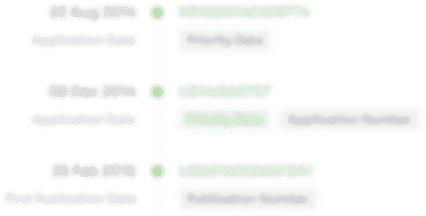
- R&D Engineer
- R&D Manager
- IP Professional
- Industry Leading Data Capabilities
- Powerful AI technology
- Patent DNA Extraction
Browse by: Latest US Patents, China's latest patents, Technical Efficacy Thesaurus, Application Domain, Technology Topic, Popular Technical Reports.
© 2024 PatSnap. All rights reserved.Legal|Privacy policy|Modern Slavery Act Transparency Statement|Sitemap|About US| Contact US: help@patsnap.com