Semi-supervised polarized SAR image classification method based on random forest composition
A technology of random forest and classification method, applied in the field of image processing, can solve the problems of not considering the spatial information of image sample points, poor classification effect, and inability to accurately represent the structure relationship of SAR data, so as to reduce the use of labeled samples, The effect of similarity relationship graph affinity and improving classification accuracy
- Summary
- Abstract
- Description
- Claims
- Application Information
AI Technical Summary
Problems solved by technology
Method used
Image
Examples
Embodiment 1
[0026] Due to the development of remote sensing technology, it has been widely used in environmental monitoring, earth resource survey, military system and other fields, and the demand for polarimetric SAR image processing is also increasing. Some existing polarimetric SAR classification methods include supervised Classification methods, the classification accuracy rate is low, and there are some graph-based semi-supervised methods, mostly by calculating the distance between image sample points to construct a similarity graph matrix, but polarimetric SAR data is nonlinear, this The composition method similarity relationship is not accurate. Therefore, the present invention proposes a semi-supervised classification method for polarization SAR images based on random forest composition, see figure 1 , including the following steps:
[0027](1) Input image: Get the raw data of the polarimetric SAR image from the polarimetric SAR image data folder. In practical applications, use ...
Embodiment 2
[0038] The polarized SAR image semi-supervised classification method based on random forest composition is the same as embodiment 1, and the process of training semi-supervised random forest in step (4) of the present invention is as follows:
[0039] 4a. Initialize the number of training iterations S=0, select s label samples from the label samples to train two classifiers respectively, the value of s depends on the actual size of the image, in this embodiment s=80;
[0040] 4b. Use the first attribute set X 1 The first KNN classifier f is trained on 80 labeled samples selected from 1 , with the second attribute set X 2 The 80 label samples selected in the training second KNN classifier f 2 ;
[0041] 4c. Use these two classifiers to assist the random forest model in semi-supervised training. The use of two classifiers to assist the random forest model in training is to ensure the efficiency of training, which is more efficient and accurate than training with one classifi...
Embodiment 3
[0044] The polarized SAR image semi-supervised classification method based on random forest composition is the same as embodiment 1-2, and the process of optimizing semi-supervised random forest in step (5) is as follows:
[0045] 5a. The present invention uses a deterministic annealing process (Deterministic Annealing process, DA) to perform optimization processing, by introducing a class label distribution probability of unlabeled data in Adjusted for normalization. Add the part of unlabeled data to the optimization goal, the expression is as follows:
[0046]
[0047] The first part of the sum term For the labeled data loss, the second term For the expectation of unlabeled data loss, the third term Expressed as the information entropy of the unlabeled data distribution; α is the expected weight value of the unlabeled data loss and α∈[0,1], the value is 0.5 in this embodiment, T is the temperature variable of annealing, in this embodiment The initial value in is...
PUM
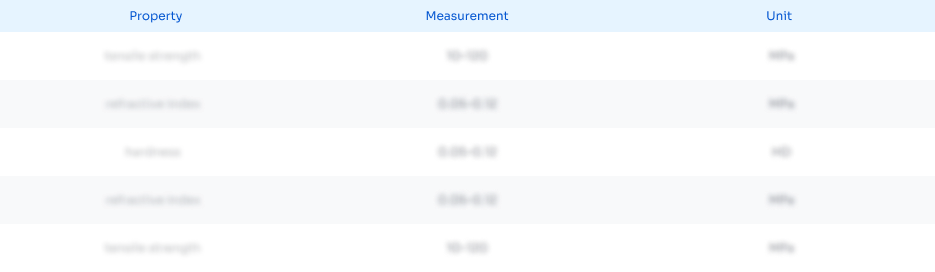
Abstract
Description
Claims
Application Information
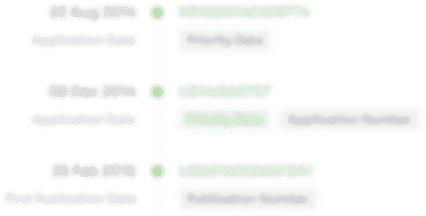
- Generate Ideas
- Intellectual Property
- Life Sciences
- Materials
- Tech Scout
- Unparalleled Data Quality
- Higher Quality Content
- 60% Fewer Hallucinations
Browse by: Latest US Patents, China's latest patents, Technical Efficacy Thesaurus, Application Domain, Technology Topic, Popular Technical Reports.
© 2025 PatSnap. All rights reserved.Legal|Privacy policy|Modern Slavery Act Transparency Statement|Sitemap|About US| Contact US: help@patsnap.com