Depth learning target detection method based on pre-segmentation and regression
A technology of target detection and deep learning, which is applied in the field of deep learning target detection, can solve the problems of poor target position positioning of the input image size, long time-consuming positioning, loss of image details, etc., to reduce the amount of calculation and calculation time, improve calculation efficiency, Avoid less robust effects
- Summary
- Abstract
- Description
- Claims
- Application Information
AI Technical Summary
Problems solved by technology
Method used
Image
Examples
Embodiment Construction
[0025] refer to figure 1 , the implementation steps of the present invention are as follows:
[0026] Step 1, establish a deep learning network model based on pre-segmentation and regression.
[0027] The current target detection network based on deep learning is divided into two categories: one is the deep learning target detection network based on candidate regions, such as R-CNN, Fast R-CNN and Faster R-CNN; the other is regression-based depth Learning target detection network, such as YOLO and SSD, the present invention proposes a deep learning target detection method based on pre-segmentation and regression. Currently, methods for extracting ROIs include: ROI extraction methods based on thresholds, ROI extraction methods based on edge extraction, ROI extraction methods based on quadtree segmentation, ROI extraction methods based on region growth, etc. , in the present invention, the region of interest extraction method using quadtree segmentation is used to construct a ...
PUM
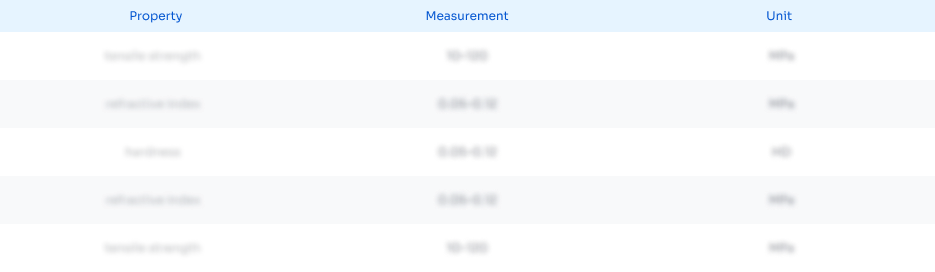
Abstract
Description
Claims
Application Information
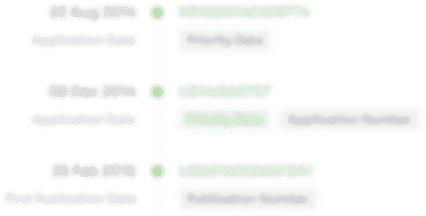
- R&D
- Intellectual Property
- Life Sciences
- Materials
- Tech Scout
- Unparalleled Data Quality
- Higher Quality Content
- 60% Fewer Hallucinations
Browse by: Latest US Patents, China's latest patents, Technical Efficacy Thesaurus, Application Domain, Technology Topic, Popular Technical Reports.
© 2025 PatSnap. All rights reserved.Legal|Privacy policy|Modern Slavery Act Transparency Statement|Sitemap|About US| Contact US: help@patsnap.com