Graph defect recognizing and positioning method based on latent Dirichlet allocation model
A technique of implicit Dirichlet and distribution models, applied in character and pattern recognition, image enhancement, image analysis, etc., can solve the problem of heavy pixel time series, and achieve high recognition rate, accurate recognition and positioning effect
- Summary
- Abstract
- Description
- Claims
- Application Information
AI Technical Summary
Problems solved by technology
Method used
Image
Examples
Embodiment Construction
[0031] The specific implementation manners of the present invention will be further described in detail below in conjunction with the accompanying drawings and embodiments. The following examples are used to illustrate the present invention, but are not intended to limit the scope of the present invention.
[0032] Refer to attached figure 1 to attach Figure 6 , the method for identifying and locating graphic defects based on the hidden Dirichlet distribution model in this embodiment. Before describing the method, first briefly introduce the calculation method of the chaotic eigenvector and the hidden Dirichlet distribution analysis model:
[0033] The literature (F.Taken, Detecting Strange Attractors in Turbulence, Lecture Notes in Mathematics, ed D.A.Rand & L.S.Young, (1981).) points out that the one-dimensional space x(t)=[x 1 (t),x 2 (t),...,x n (t)]∈R n It can be mapped to m-dimensional space, the process is as follows:
[0034]
[0035]
[0036] Here, τ rep...
PUM
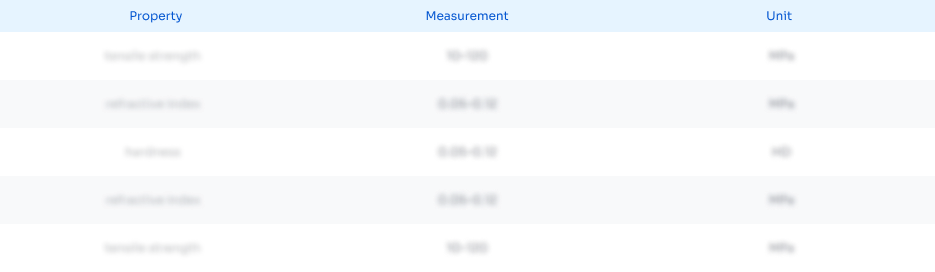
Abstract
Description
Claims
Application Information
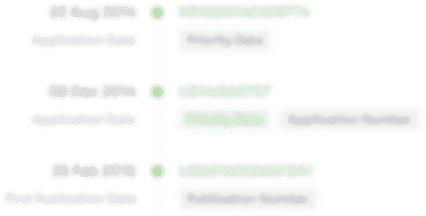
- R&D Engineer
- R&D Manager
- IP Professional
- Industry Leading Data Capabilities
- Powerful AI technology
- Patent DNA Extraction
Browse by: Latest US Patents, China's latest patents, Technical Efficacy Thesaurus, Application Domain, Technology Topic, Popular Technical Reports.
© 2024 PatSnap. All rights reserved.Legal|Privacy policy|Modern Slavery Act Transparency Statement|Sitemap|About US| Contact US: help@patsnap.com