Moving workpiece recognition method based on spatiotemporal contexts and fully convolutional network
A spatiotemporal context, fully convolutional network technology, applied in the field of digital image processing target detection and recognition, to improve the degree of intelligence and achieve the effect of semantic segmentation and classification
- Summary
- Abstract
- Description
- Claims
- Application Information
AI Technical Summary
Problems solved by technology
Method used
Image
Examples
Embodiment 1
[0058] Embodiment 1: as Figure 1-9 As shown, the moving workpiece recognition method based on the spatio-temporal context full convolutional network, firstly, use the target image database (5 kinds of common machinery industry tools and workpieces: bearings, screwdrivers, gears, pliers, wrenches) to carry out the full convolutional neural network Train the target classifier to be classified; then, use the background difference method and digital image processing morphology method to obtain the initial position of the target in the first frame of the video sequence, and use the space-time context model target tracking method to track the target to be tracked according to the initial position. The accuracy map verifies the target tracking accuracy; finally, the tracked results are classified and identified using the trained classifier to achieve semantic segmentation, thereby obtaining the target category. Verification of Semantic Classification Recognition Performance by Groun...
PUM
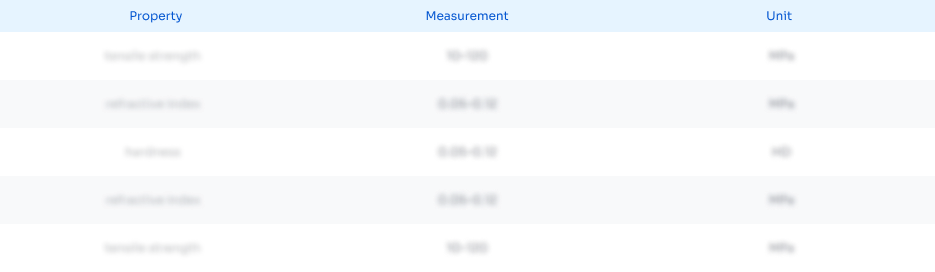
Abstract
Description
Claims
Application Information
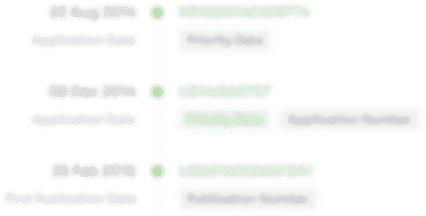
- R&D Engineer
- R&D Manager
- IP Professional
- Industry Leading Data Capabilities
- Powerful AI technology
- Patent DNA Extraction
Browse by: Latest US Patents, China's latest patents, Technical Efficacy Thesaurus, Application Domain, Technology Topic, Popular Technical Reports.
© 2024 PatSnap. All rights reserved.Legal|Privacy policy|Modern Slavery Act Transparency Statement|Sitemap|About US| Contact US: help@patsnap.com