Face super-resolution reconstruction method based on feature transformation based on nearest feature line
A recent feature line and feature conversion technology, applied in the field of image processing, can solve the problems of training sample library size limitation, inability to meet noise robustness, and unsatisfactory reconstruction effect
- Summary
- Abstract
- Description
- Claims
- Application Information
AI Technical Summary
Problems solved by technology
Method used
Image
Examples
Embodiment Construction
[0051] The technical solution of the present invention can adopt the form of software to realize automatic process operation. The technical solution of the present invention will be further elaborated below in conjunction with the embodiments and accompanying drawings, as follows: figure 1 , figure 2 and image 3 As shown, a face super-resolution reconstruction method based on feature transformation based on the nearest feature line, specifically includes the following steps:
[0052] Step 1, input low-resolution face image x to be reconstructed, low-resolution image training sample set and high-resolution image training sample set N represents the number of training sample face patterns in the low-resolution image training sample set X and the high-resolution image training sample set Y.
[0053] In this embodiment, the FEI face database in the face super-resolution field is selected as the training sample library for the algorithm reconstruction experiment; the FEI fac...
PUM
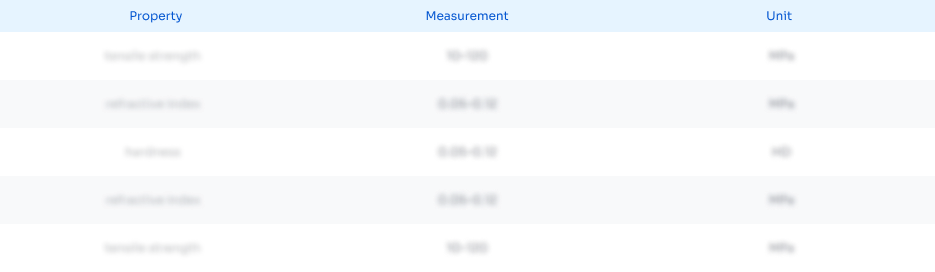
Abstract
Description
Claims
Application Information
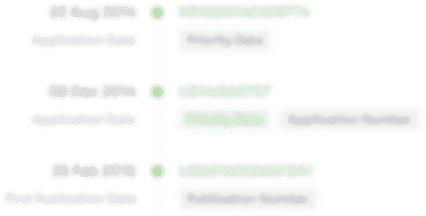
- R&D Engineer
- R&D Manager
- IP Professional
- Industry Leading Data Capabilities
- Powerful AI technology
- Patent DNA Extraction
Browse by: Latest US Patents, China's latest patents, Technical Efficacy Thesaurus, Application Domain, Technology Topic, Popular Technical Reports.
© 2024 PatSnap. All rights reserved.Legal|Privacy policy|Modern Slavery Act Transparency Statement|Sitemap|About US| Contact US: help@patsnap.com