Method for judging willingness-to-pay
A technology of willingness to pay and influencing factors, applied in the field of judging willingness to pay and judging willingness to pay based on a Bayesian network model, can solve the problems of inability to repeat predictions, reduce prediction accuracy, and ignore interrelationships, so as to improve authenticity and scientific Sexuality, avoid subjectivity and poor inhibition, improve the effect of precision
- Summary
- Abstract
- Description
- Claims
- Application Information
AI Technical Summary
Problems solved by technology
Method used
Image
Examples
Embodiment 1
[0047] Example 1 Obtaining influencing factors related to willingness to pay
[0048] (1) Collect the social characteristics and willingness to pay information of different groups of people through questionnaires. The questionnaire on the service value of the Beijing South-to-North Water Diversion Water Resources Reserve Action consists of three parts, which are the social characteristics of the respondents, the respondents' understanding of the survey background, and the respondents' willingness to pay. The first part is to collect relevant social background information of the respondents, including age, gender, education, income, occupation, housing type and other six questions; Survey to understand the situation; the third part is to obtain the respondent's willingness to pay, that is, the personal WTP value.
[0049] A total of 906 sample data were received, 776 were valid data, and the effective rate was 85.7%. The population of this survey covers different genders, age...
Embodiment 2
[0064] Example 2 Constructing a Bayesian Network Model of Willingness to Pay
[0065] (1) Classify the three influencing factors directly related to the willingness to pay as the first level of the Bayesian network structure; classify the factors that affect the first level as the second level, and establish a Bayesian network structure diagram, such as figure 1 shown. It can be seen from the figure that one variable can affect two sub-nodes at the same time. For example, the change of the age (AG) node can not only affect the monthly family income (FI) of the node, but also affect the public's approval of the node for the project to spend manpower, material and financial resources to reserve water resources. degree (EC), participation in public welfare activities (CW). Based on the Bayesian network, it can comprehensively display the influence of different factors on the willingness to pay and the relationship between them.
[0066] (2) The conditional probabilities of most...
Embodiment 3
[0072] Example 3 Sensitivity Analysis Affecting Willingness to Pay
[0073] Sensitivity analysis using Bayesian network can find out the key factors affecting willingness to pay. By changing the probability table of the selected node variable, the change of the probability table of the target node variable can be seen. The importance of the selected node variable SN to the target node variable TN is expressed by the importance index I:
[0074]
[0075] Among them, P(TN) is the prior probability of the target node variable TN, and P(TN|SN) is the conditional probability of the target node TN under the change of the selected node variable SN. The importance index I reflects the degree of influence of the selected node variables on the target node, and the important factors affecting the willingness to pay can be obtained by comparing the size of I.
[0076] This paper selects the variable state with the largest importance index I among the variables of each node, and the t...
PUM
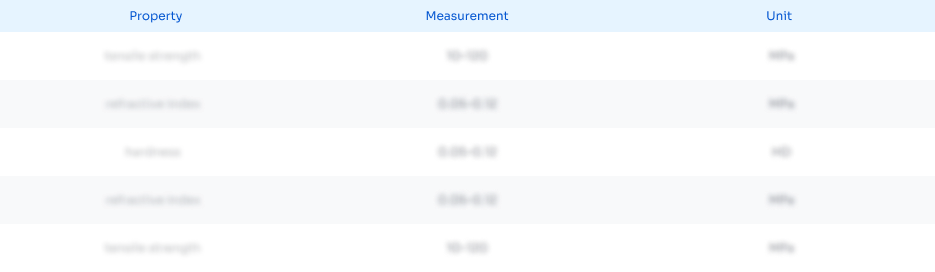
Abstract
Description
Claims
Application Information
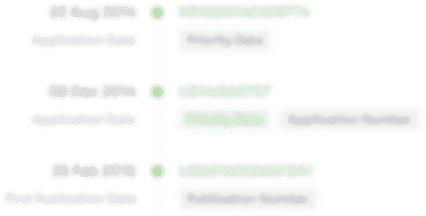
- R&D Engineer
- R&D Manager
- IP Professional
- Industry Leading Data Capabilities
- Powerful AI technology
- Patent DNA Extraction
Browse by: Latest US Patents, China's latest patents, Technical Efficacy Thesaurus, Application Domain, Technology Topic, Popular Technical Reports.
© 2024 PatSnap. All rights reserved.Legal|Privacy policy|Modern Slavery Act Transparency Statement|Sitemap|About US| Contact US: help@patsnap.com