Video monitoring equipment fault automatic detection method
A technology for video surveillance and equipment failure, applied in the field of automatic detection of video surveillance equipment failure, can solve problems such as reducing computing efficiency, unable to effectively solve image data pooling and whitening, etc.
- Summary
- Abstract
- Description
- Claims
- Application Information
AI Technical Summary
Problems solved by technology
Method used
Image
Examples
Embodiment Construction
[0021] In order to make the object, technical solution and advantages of the present invention more clear, the present invention will be further described in detail below in conjunction with the accompanying drawings and embodiments. It should be understood that the specific embodiments described here are only used to explain the present invention, not to limit the present invention.
[0022] In the embodiment of the present invention, a method for automatic detection of video surveillance equipment faults is provided, such as figure 1 As shown, step 1, the convolutional self-encoder uses different network layers and hidden node numbers to extract the features of the monitoring image, performs image feature extraction in the pooling layer, and iterates the convolutional self-encoder until the accuracy of the classification model converges; step Second, the convolutional neural network uses the features of the surveillance image extracted by the convolutional self-encoder as th...
PUM
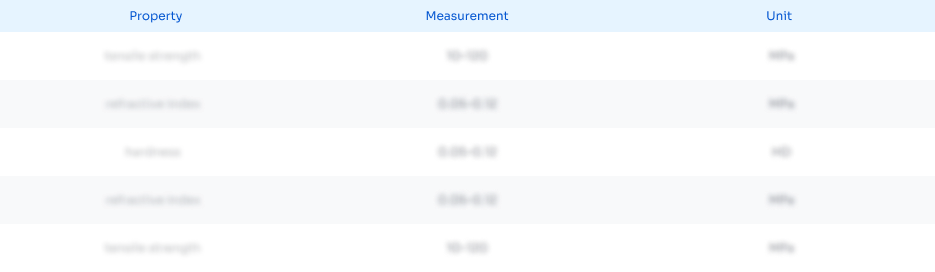
Abstract
Description
Claims
Application Information
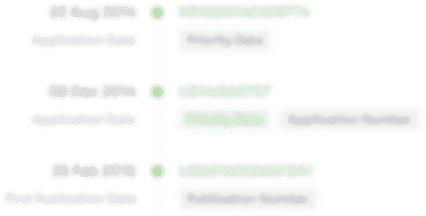
- R&D
- Intellectual Property
- Life Sciences
- Materials
- Tech Scout
- Unparalleled Data Quality
- Higher Quality Content
- 60% Fewer Hallucinations
Browse by: Latest US Patents, China's latest patents, Technical Efficacy Thesaurus, Application Domain, Technology Topic, Popular Technical Reports.
© 2025 PatSnap. All rights reserved.Legal|Privacy policy|Modern Slavery Act Transparency Statement|Sitemap|About US| Contact US: help@patsnap.com