Complex device performance evaluating and predicting method of multi-source no-label data machine learning
A machine learning and prediction method technology, applied in the direction of electrical digital data processing, special data processing applications, instruments, etc., can solve the problems that complex equipment is difficult to work, ignores the optimization of model structure, and lacks consideration of the nonlinear dominant factors of complex equipment. To achieve the effect of ensuring effective implementation and high solution accuracy
- Summary
- Abstract
- Description
- Claims
- Application Information
AI Technical Summary
Problems solved by technology
Method used
Image
Examples
Embodiment Construction
[0039] The present invention provides a complex equipment performance evaluation and prediction method for multi-source unlabeled data machine learning, using Autoencoder to realize non-supervised fusion dimensionality reduction processing of multi-source unlabeled degraded data, and obtain comprehensive degradation features representing the performance of complex equipment; The non-homogeneous hidden semi-Markov model with structure and parameter optimization obtains the initial value of the hidden performance state of the aeroengine, the change moment and the duration of each state, and finally realizes performance evaluation and remaining service life prediction. This method not only overcomes the dependence of performance evaluation and prediction on degradation prior knowledge and health state labels, fully considers the nonlinear dominant factors of complex equipment, but also solves the problem that the traditional non-supervised method needs to specify the number of hidd...
PUM
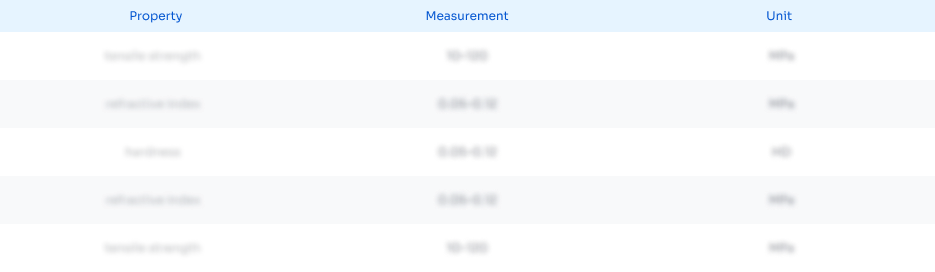
Abstract
Description
Claims
Application Information
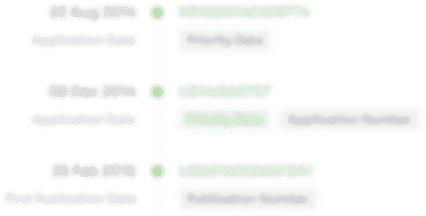
- R&D
- Intellectual Property
- Life Sciences
- Materials
- Tech Scout
- Unparalleled Data Quality
- Higher Quality Content
- 60% Fewer Hallucinations
Browse by: Latest US Patents, China's latest patents, Technical Efficacy Thesaurus, Application Domain, Technology Topic, Popular Technical Reports.
© 2025 PatSnap. All rights reserved.Legal|Privacy policy|Modern Slavery Act Transparency Statement|Sitemap|About US| Contact US: help@patsnap.com