Multi-discriminator error back propagation confrontation network method
An error backpropagation and discriminator technology, applied in the field of deep learning neural network, can solve problems such as deviation, affecting the accuracy and speed of network training, and achieve the effect of stable training, feasibility, and enhanced objectivity
- Summary
- Abstract
- Description
- Claims
- Application Information
AI Technical Summary
Problems solved by technology
Method used
Image
Examples
Embodiment
[0030] This embodiment discloses an adversarial network method for multi-discriminator error backpropagation, which specifically includes the following steps:
[0031] Step S1, constructing a generative confrontation network GAN model, the model includes a generator and a discriminator;
[0032] Step S2, constructing multiple discriminators on the basis of the existing GAN model;
[0033] Step S3, preparing the data set to train the improved multi-discriminator network.
[0034] According to the number of discriminators, the same number of real images in the selected data set will be different from the real images in the data set and input into the discriminator for training. The result of such a training method is that different discriminators receive different real images in the data set, and after training, the parameter weights of each discriminator are also different. Using multiple discriminators with different parameter weights to judge the generated image, the angle ...
PUM
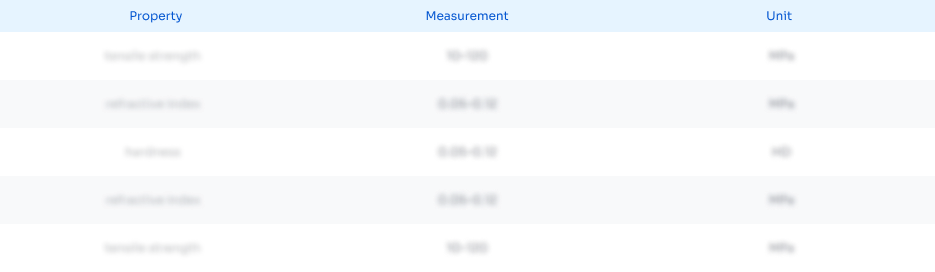
Abstract
Description
Claims
Application Information
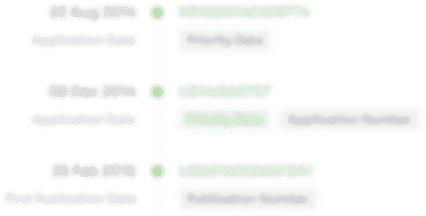
- Generate Ideas
- Intellectual Property
- Life Sciences
- Materials
- Tech Scout
- Unparalleled Data Quality
- Higher Quality Content
- 60% Fewer Hallucinations
Browse by: Latest US Patents, China's latest patents, Technical Efficacy Thesaurus, Application Domain, Technology Topic, Popular Technical Reports.
© 2025 PatSnap. All rights reserved.Legal|Privacy policy|Modern Slavery Act Transparency Statement|Sitemap|About US| Contact US: help@patsnap.com