An inductive non-negative projection semi-supervised data classification method and system
A data classification and semi-supervised technology, applied in character and pattern recognition, instruments, calculations, etc., can solve various problems such as noise, classification performance impact, data redundancy, etc., achieve accurate data representation results, maintain neighborhood information and Spatial structure and good expansion performance
- Summary
- Abstract
- Description
- Claims
- Application Information
AI Technical Summary
Problems solved by technology
Method used
Image
Examples
Embodiment 1
[0034] The present invention is tested on 6 real data sets, including MIT face, AR male and female face, YaleB face, ORL face and Yale face. Based on the consideration of computational efficiency, the size of all real images is compressed to 32x32; in the experiment, each image corresponds to a 1024-dimensional vector. In the experiment, any number of each class is randomly selected from each data set as labeled samples, and any number of each class is randomly selected as unlabeled samples. These datasets were collected from multiple sources, so the test results are generally descriptive.
[0035] see figure 1 As shown, an inductive non-negative projection semi-supervised data classification method includes the following steps:
[0036] (1), randomly divide the original data set into a training set and a test set, then initialize the training set and the test set to obtain an initial category label matrix;
[0037] Step (1) specifically includes:
[0038]The original samp...
PUM
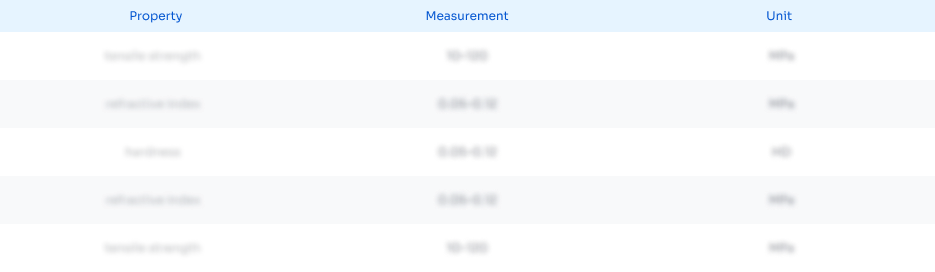
Abstract
Description
Claims
Application Information
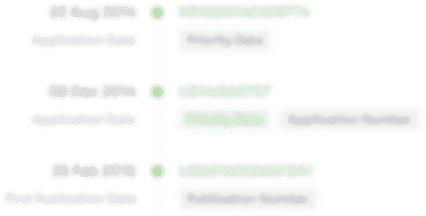
- R&D
- Intellectual Property
- Life Sciences
- Materials
- Tech Scout
- Unparalleled Data Quality
- Higher Quality Content
- 60% Fewer Hallucinations
Browse by: Latest US Patents, China's latest patents, Technical Efficacy Thesaurus, Application Domain, Technology Topic, Popular Technical Reports.
© 2025 PatSnap. All rights reserved.Legal|Privacy policy|Modern Slavery Act Transparency Statement|Sitemap|About US| Contact US: help@patsnap.com