Recurrent neural network sparse connection method based on block tensor decomposition
A technology of cyclic neural network and tensor decomposition, applied in neural learning methods, biological neural network models, etc., can solve problems such as ignoring high-dimensional facts and redundant characteristics of full connections, and achieve the goal of improving sharing and training speed Effect
- Summary
- Abstract
- Description
- Claims
- Application Information
AI Technical Summary
Problems solved by technology
Method used
Image
Examples
Embodiment Construction
[0024] In order to facilitate those skilled in the art to understand the technical content of the present invention, the content of the present invention will be further explained below in conjunction with the accompanying drawings.
[0025] like figure 1 Shown is the scheme flowchart of the present invention, and the technical scheme of the present invention is: the cyclic neural network sparse connection method based on block tensor decomposition, comprising:
[0026] S1. Tensorize the input vector x of the network to obtain the first tensor Quantize the memory vector h to get the second tensor Quantize the fully connected weight matrix W to get the third tensor
[0027] Suppose the input vector memory vector Fully connected weight matrix constructed tensor and is a d-dimensional tensor, is a 2d-dimensional tensor, where I=I 1 ·I 2 ·...·I d , J=J 1 ·J 2 ·...·J d . The tensorization operation in the present invention refers to rearranging the elements...
PUM
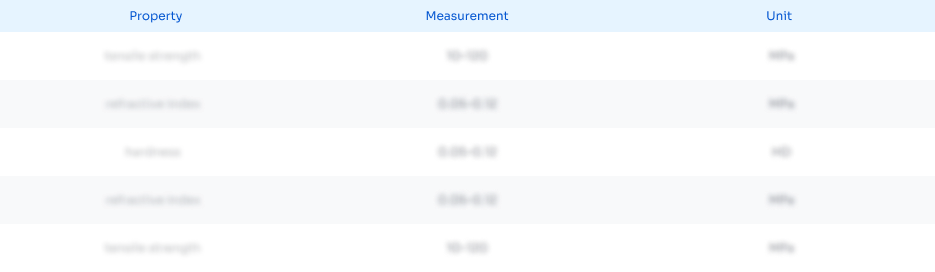
Abstract
Description
Claims
Application Information
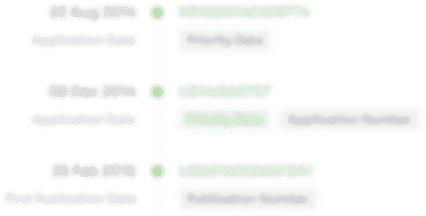
- R&D Engineer
- R&D Manager
- IP Professional
- Industry Leading Data Capabilities
- Powerful AI technology
- Patent DNA Extraction
Browse by: Latest US Patents, China's latest patents, Technical Efficacy Thesaurus, Application Domain, Technology Topic, Popular Technical Reports.
© 2024 PatSnap. All rights reserved.Legal|Privacy policy|Modern Slavery Act Transparency Statement|Sitemap|About US| Contact US: help@patsnap.com