Semi-supervised-transfer-learning character recognition method and system based on convolutional neural network
A convolutional neural network and transfer learning technology, applied in neural learning methods, character recognition, character and pattern recognition, etc., can solve the problems of affecting recognition accuracy, difficult to obey statistical distribution, and low sample adaptability, and achieve high recognition accuracy. , the effect of improving adaptability
- Summary
- Abstract
- Description
- Claims
- Application Information
AI Technical Summary
Problems solved by technology
Method used
Image
Examples
Embodiment Construction
[0045] The specific implementation manners of the present invention will be further described in detail below in conjunction with the accompanying drawings and embodiments. The following examples are used to illustrate the present invention, but are not intended to limit the scope of the present invention.
[0046] figure 1 It is a flow chart of a semi-supervised transfer learning character recognition method based on a convolutional neural network provided by an embodiment of the present invention, such as figure 1 As shown, the method includes:
[0047] Step 1, using batches of character image samples without class labels in the target domain as a test sample set input to the convolutional neural network after semi-supervised transfer learning, and identifying the character images of the test sample set;
[0048] The convolutional neural network after the semi-supervised transfer learning consists of a batch of character image samples with class labels in the source domain, ...
PUM
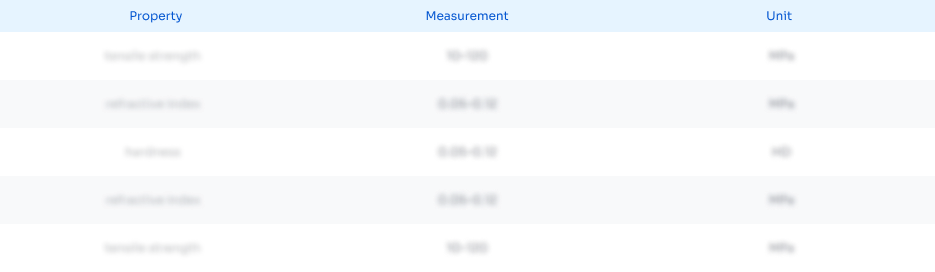
Abstract
Description
Claims
Application Information
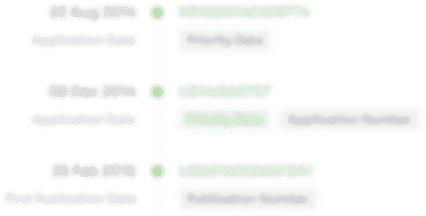
- Generate Ideas
- Intellectual Property
- Life Sciences
- Materials
- Tech Scout
- Unparalleled Data Quality
- Higher Quality Content
- 60% Fewer Hallucinations
Browse by: Latest US Patents, China's latest patents, Technical Efficacy Thesaurus, Application Domain, Technology Topic, Popular Technical Reports.
© 2025 PatSnap. All rights reserved.Legal|Privacy policy|Modern Slavery Act Transparency Statement|Sitemap|About US| Contact US: help@patsnap.com