Echocardiographic ventricle segmentation method and device based on deep learning and deformation model
A technology of echocardiography and deformation models, which is applied in the field of medical image processing, can solve the problems of large manpower, material resources, and negative effects on the calculation of related indicators of the ventricle, and achieve the effect of saving working time
- Summary
- Abstract
- Description
- Claims
- Application Information
AI Technical Summary
Problems solved by technology
Method used
Image
Examples
specific Embodiment approach 1
[0034] Specific Embodiment 1: The echocardiographic ventricle segmentation method based on deep learning and deformation model of this embodiment, such as figure 1 shown, including the following steps:
[0035] Step 1: Obtain hypercardiographic data.
[0036] Step 2. Manually mark the endocardium of the ventricles in the hypercardiogram data.
[0037] Step 3: Preprocessing the images marked with the endocardium of the ventricle as training data.
[0038] Step 4: Using the training data to train the rough segmentation model of the ventricle, and obtain the rough segmentation training result.
[0039] Step 5. Calculate the center point of the rough segmentation training result in each section, fit a straight line according to all the center points, and calculate the distance between all the center points and the outer edge of the rough segmentation training result in the direction perpendicular to the straight line Average value as radius.
[0040] Step 6: Perform resampling...
Embodiment approach
[0044] 1. Clinical acquisition of echocardiographic data
[0045] figure 2 is the echocardiographic short-axis tangential basal image of the ventricle, image 3 is the echocardiographic short-axis tangential mid-portion image of the ventricle, Figure 4 is the echocardiographic ventricular short-axis tangential apical image, Figure 5 is the echocardiographic long-axis "four-chamber heart" slice image, Figure 6 is the echocardiographic long-axis "two-chamber heart" slice image, Figure 7 It's a three-dimensional echocardiogram. according to figure 2 As shown in , 3, 4, 5, 6 or 7, two-dimensional data or three-dimensional echocardiographic data of each measured person is clinically collected. In order to train a more accurate coarse segmentation model, the number of samples of the tested personnel should be kept above 500 as much as possible. Different tangential data are used as input to provide the heart information of the person being tested, so as to achieve a mor...
specific Embodiment approach 2
[0066] Embodiment 2: This embodiment differs from Embodiment 1 in that: the echocardiographic data is two-dimensional, multi-phase and multi-directional echocardiographic slice data or three-dimensional echocardiographic data acquired through ultrasonic equipment.
[0067] Other steps and parameters are the same as those in Embodiment 1.
PUM
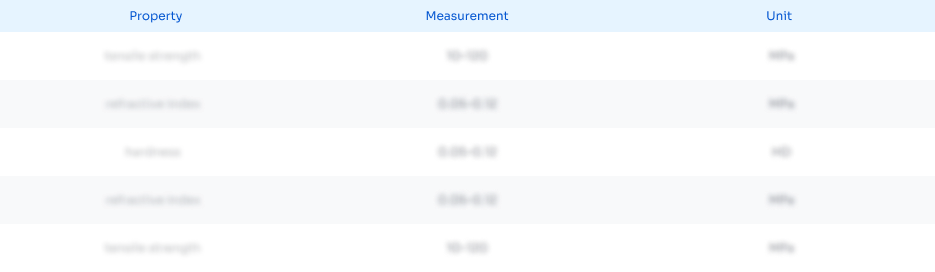
Abstract
Description
Claims
Application Information
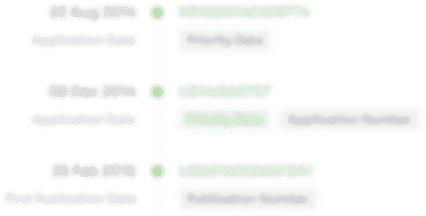
- R&D Engineer
- R&D Manager
- IP Professional
- Industry Leading Data Capabilities
- Powerful AI technology
- Patent DNA Extraction
Browse by: Latest US Patents, China's latest patents, Technical Efficacy Thesaurus, Application Domain, Technology Topic, Popular Technical Reports.
© 2024 PatSnap. All rights reserved.Legal|Privacy policy|Modern Slavery Act Transparency Statement|Sitemap|About US| Contact US: help@patsnap.com