Weak and small object recognition method based on deep neural network
A deep neural network and weak target technology, applied in neural learning methods, biological neural network models, character and pattern recognition, etc., can solve problems such as low accuracy, complex structure, underfitting of weak target recognition, etc., and achieve salience Improved and accurate classification effect
- Summary
- Abstract
- Description
- Claims
- Application Information
AI Technical Summary
Problems solved by technology
Method used
Image
Examples
Embodiment Construction
[0016] See figure 1 , the deep neural network structure proposed by the present invention has a total of 23 layers, of which 21 layers are hidden layers, including 5 layers of convolutional layers, 3 layers of fully connected layers, 7 layers of ReLU layers, 3 layers of normalization layers and 3 pooling layers layer. The input of the neural network is a 65×65 RGB color image, and the output is two probability values, which respectively represent the probability that the input image is the target and not the target.
[0017] The data accepted by the input layer of the deep neural network is an RGB color image of 65×65 pixels. The convolution kernel size of the first convolutional layer is 5×5×3, a total of 64, and the convolution operation with a step size of 2 is performed. , and then after a pooling layer with a step size of 2 and a size of 3×3 pixels and a regularization layer, a set of data with a size of 17×17×64 is output.
[0018] The second convolutional layer is com...
PUM
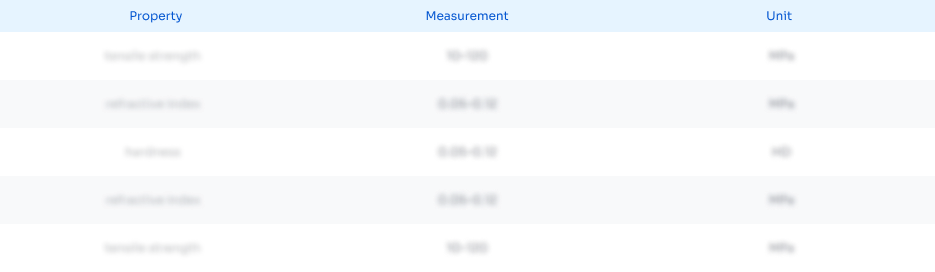
Abstract
Description
Claims
Application Information
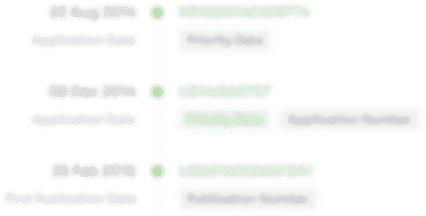
- R&D Engineer
- R&D Manager
- IP Professional
- Industry Leading Data Capabilities
- Powerful AI technology
- Patent DNA Extraction
Browse by: Latest US Patents, China's latest patents, Technical Efficacy Thesaurus, Application Domain, Technology Topic, Popular Technical Reports.
© 2024 PatSnap. All rights reserved.Legal|Privacy policy|Modern Slavery Act Transparency Statement|Sitemap|About US| Contact US: help@patsnap.com