Method for achieving robot multi-axis-hole assembling through deep reinforcement learning
A technology of reinforcement learning and robotics, applied in manipulators, program-controlled manipulators, manufacturing tools, etc., can solve problems such as poor versatility, inaccurate analysis of contact state jamming models, and poor generalization ability
- Summary
- Abstract
- Description
- Claims
- Application Information
AI Technical Summary
Problems solved by technology
Method used
Image
Examples
Embodiment Construction
[0066]The method proposed by the present invention utilizes deep reinforcement learning to realize robot multi-axis hole assembly, and its flow chart is as follows figure 1 As shown, including establishing a simulation model, using the established simulation model to generate an empirical data set, and using the data in the empirical data set to train the deep reinforcement learning network to complete the assembly task of the robot,
[0067] The establishment of the simulation model includes the following steps:
[0068] (1) Establish the axis three-dimensional coordinate system X-Y-Z on the shaft component to be assembled, the coordinate origin O of the three-dimensional coordinate system X-Y-Z is located at the midpoint of the line connecting the centers of the two axes on the upper surface of the axis to be assembled, and the positive direction of the Z axis is downward along the axis of the axis, The positive direction of the X-axis is along the center of the left axis p...
PUM
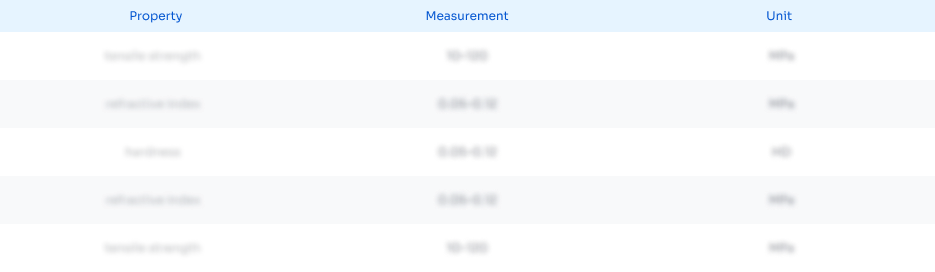
Abstract
Description
Claims
Application Information
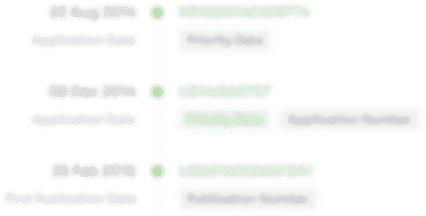
- Generate Ideas
- Intellectual Property
- Life Sciences
- Materials
- Tech Scout
- Unparalleled Data Quality
- Higher Quality Content
- 60% Fewer Hallucinations
Browse by: Latest US Patents, China's latest patents, Technical Efficacy Thesaurus, Application Domain, Technology Topic, Popular Technical Reports.
© 2025 PatSnap. All rights reserved.Legal|Privacy policy|Modern Slavery Act Transparency Statement|Sitemap|About US| Contact US: help@patsnap.com