A joint learning deep network model for optical flow estimation and denoising of video images
A deep network and video image technology, applied in the field of image processing, can solve the problems of poor denoising effect, low accuracy of optical flow estimation, and long time consumption
- Summary
- Abstract
- Description
- Claims
- Application Information
AI Technical Summary
Problems solved by technology
Method used
Image
Examples
Embodiment Construction
[0026] The following will clearly and completely describe the technical solutions in the embodiments of the present invention with reference to the accompanying drawings in the embodiments of the present invention. Obviously, the described embodiments are only some of the embodiments of the present invention, not all of them. Based on the embodiments of the present invention, all other embodiments obtained by persons of ordinary skill in the art without making creative efforts belong to the protection scope of the present invention.
[0027] Such as figure 1 As shown, this embodiment provides a joint learning deep network model for optical flow estimation and denoising of video images, including three modules: a preprocessing module, an optical flow estimation module and a denoising module. The sample data set, where each sample includes two adjacent frames of noise images n1 and n2 in the video, such as figure 2 As shown, noise images n1 and n2 correspond to standard defini...
PUM
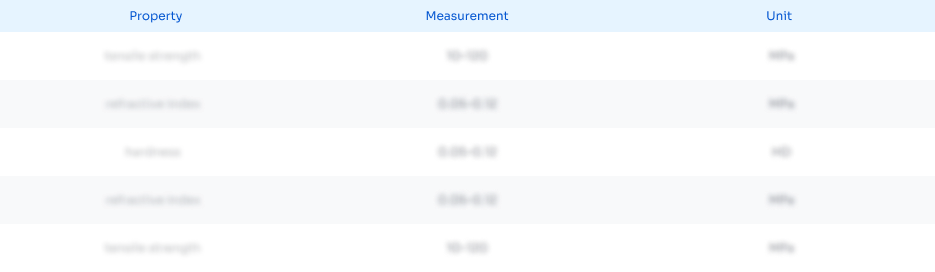
Abstract
Description
Claims
Application Information
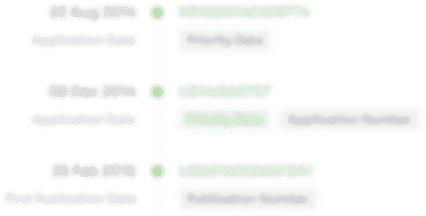
- R&D Engineer
- R&D Manager
- IP Professional
- Industry Leading Data Capabilities
- Powerful AI technology
- Patent DNA Extraction
Browse by: Latest US Patents, China's latest patents, Technical Efficacy Thesaurus, Application Domain, Technology Topic, Popular Technical Reports.
© 2024 PatSnap. All rights reserved.Legal|Privacy policy|Modern Slavery Act Transparency Statement|Sitemap|About US| Contact US: help@patsnap.com