Cost-sensitive stacking integrated learning framework based on feature inverse mapping
A cost-sensitive, integrated learning technology, applied in the field of pattern recognition, to achieve the effect of reducing deviation, reducing time complexity, and saving parameter selection time
- Summary
- Abstract
- Description
- Claims
- Application Information
AI Technical Summary
Problems solved by technology
Method used
Image
Examples
Embodiment Construction
[0011] Below in conjunction with accompanying drawing and example the present invention will be further introduced: the system designed by the present invention is divided into four modules altogether.
[0012] Part I: Data Acquisition
[0013] The process of data collection is to convert real samples into data, and generate a data set represented by vectors for subsequent modules to process. In this step, the collected samples are divided into training samples and testing samples. The training samples are processed first. A training sample generates a vector Among them, i indicates that the sample is the i-th of the total training samples, and c indicates that the sample belongs to the c-th class. Each element of the vector corresponds to an attribute of the sample, and the dimension d of the vector is the number of attributes of the sample. For the convenience of subsequent calculations, all training samples are combined into a training matrix D, in which each row is a ...
PUM
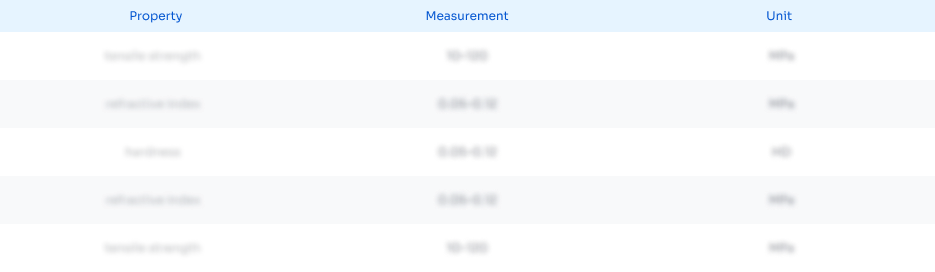
Abstract
Description
Claims
Application Information
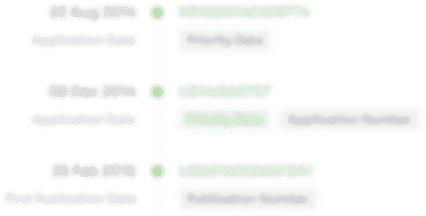
- R&D Engineer
- R&D Manager
- IP Professional
- Industry Leading Data Capabilities
- Powerful AI technology
- Patent DNA Extraction
Browse by: Latest US Patents, China's latest patents, Technical Efficacy Thesaurus, Application Domain, Technology Topic, Popular Technical Reports.
© 2024 PatSnap. All rights reserved.Legal|Privacy policy|Modern Slavery Act Transparency Statement|Sitemap|About US| Contact US: help@patsnap.com