A Fault Diagnosis Technology of Mechanical Bearing Based on Wide Residual Network Learning Model
A fault diagnosis and network learning technology, applied in the field of mechanical vibration, can solve problems such as increasing depth, and achieve the effect of high accuracy and feasible application
- Summary
- Abstract
- Description
- Claims
- Application Information
AI Technical Summary
Problems solved by technology
Method used
Image
Examples
Embodiment
[0055] A mechanical bearing fault diagnosis technology based on wide residual network learning model, the specific process is as follows:
[0056] 1. Collect vibration data. According to the sampling theorem, the sampling frequency is greater than twice the signal frequency. Taking the rolling element fault as an example, 1024 sampling points are selected for sampling per unit time, that is, the sampling frequency is 1024Hz>2*137.48Hz, and this sampling frequency is also consistent with other faults. In order to effectively retain the fault characteristics of the original vibration signal, a single learning sample is set to a size of 32*32 (1024 sampling points), and the vibration data is converted into a data set format of 32*32 grayscale image, through a bit depth of 8 The size of the gray value of the bit grayscale image represents the vibration amplitude of the vibration data, and then the data set is divided into a training set and a test set according to a certain ratio...
PUM
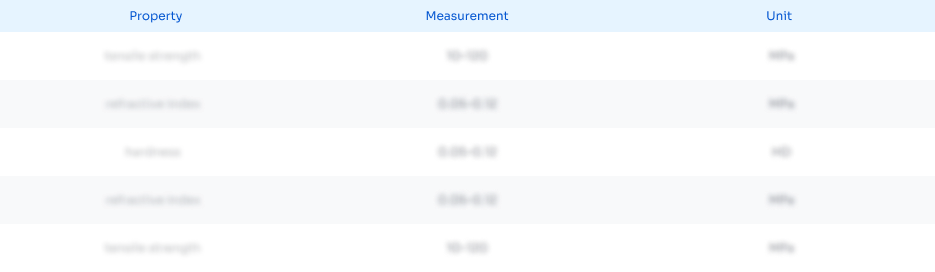
Abstract
Description
Claims
Application Information
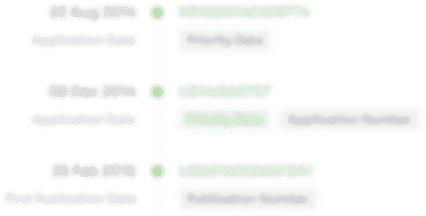
- R&D Engineer
- R&D Manager
- IP Professional
- Industry Leading Data Capabilities
- Powerful AI technology
- Patent DNA Extraction
Browse by: Latest US Patents, China's latest patents, Technical Efficacy Thesaurus, Application Domain, Technology Topic.
© 2024 PatSnap. All rights reserved.Legal|Privacy policy|Modern Slavery Act Transparency Statement|Sitemap