Self-encoding neural network-based symbol diagram node classification method
A neural network and node classification technology, applied in character and pattern recognition, instruments, data processing applications, etc., can solve the problems of not considering the node relationship in the graph, not suitable for symbol graph learning, etc., to achieve less training data and computational complexity. low effect
- Summary
- Abstract
- Description
- Claims
- Application Information
AI Technical Summary
Problems solved by technology
Method used
Image
Examples
Embodiment
[0038] A symbolic graph node classification method based on self-encoding neural network, which is used to realize the unsupervised learning of symbolic graph model node representation vectors, and can rely on a small amount of labeled data to train classifiers to realize node type classification. The input of the method is a symbolic graph structure. The output is the category of the node. In this embodiment, user classification in a social network is taken as an example to describe implementation steps of the present invention in detail. Such as figure 1 As shown, the symbol graph node classification method based on self-encoder neural network includes the following steps:
[0039] S1. Construct an adjacency matrix based on the symbolic graph structure, specifically:
[0040] Given a symbolic graph containing N nodes, construct an N×N-dimensional matrix M, the value m of row i and j in M ij Indicates the connection status of the i-th and j-th nodes in the symbol graph, if...
PUM
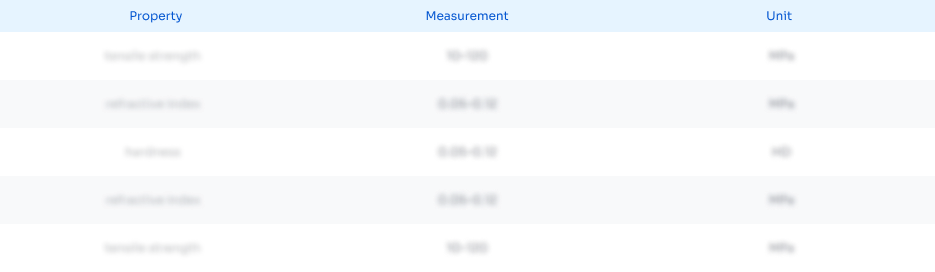
Abstract
Description
Claims
Application Information
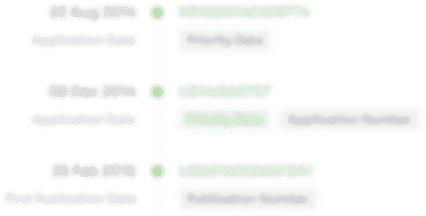
- R&D Engineer
- R&D Manager
- IP Professional
- Industry Leading Data Capabilities
- Powerful AI technology
- Patent DNA Extraction
Browse by: Latest US Patents, China's latest patents, Technical Efficacy Thesaurus, Application Domain, Technology Topic, Popular Technical Reports.
© 2024 PatSnap. All rights reserved.Legal|Privacy policy|Modern Slavery Act Transparency Statement|Sitemap|About US| Contact US: help@patsnap.com