RBF-neural-network-based atmospheric pollutant concentration prediction method
An atmospheric pollutant and neural network technology, which is applied in the field of atmospheric pollutant concentration prediction based on RBF neural network, can solve the problems of unstable central point, not fully considering the distribution of data, affecting the performance of RBF neural network, etc. Accuracy, the effect of improving prediction accuracy
- Summary
- Abstract
- Description
- Claims
- Application Information
AI Technical Summary
Problems solved by technology
Method used
Image
Examples
Embodiment Construction
[0071] The present invention will be further described below in conjunction with accompanying drawing.
[0072] A kind of air pollutant concentration prediction method based on RBF neural network of the present invention comprises the following steps:
[0073] 1) According to the actual situation of the predicted area, divide the selected experimental data, including air pollutant concentration data and weather data, and preprocess the air pollutant concentration data;
[0074] 2) For the preprocessed air pollutant concentration data, use MMOD's improved k-means++ algorithm to find the cluster centers, and calculate each kernel function based on the variance, namely Gaussian, thin plate spline and inverse multi-quadratic kernels the width of the function;
[0075] 3) Using the integrated RBFNN algorithm and using the Bagging strategy to sample the experimental data, the data subset of the RBF neural network that participated in the creation is IOB, and the remaining unsampled...
PUM
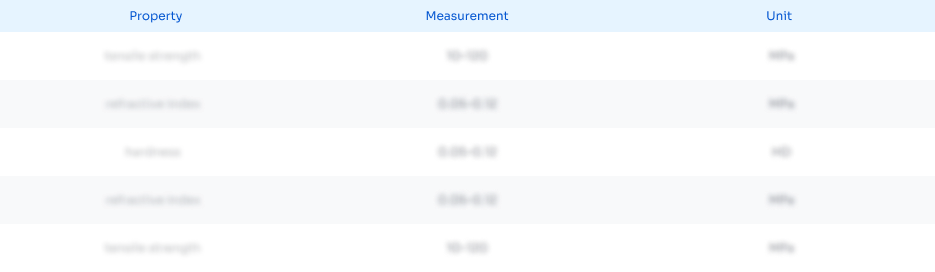
Abstract
Description
Claims
Application Information
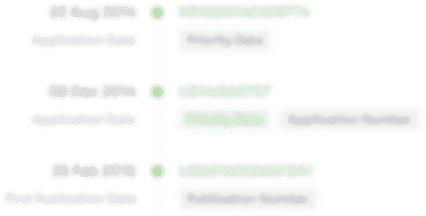
- R&D Engineer
- R&D Manager
- IP Professional
- Industry Leading Data Capabilities
- Powerful AI technology
- Patent DNA Extraction
Browse by: Latest US Patents, China's latest patents, Technical Efficacy Thesaurus, Application Domain, Technology Topic, Popular Technical Reports.
© 2024 PatSnap. All rights reserved.Legal|Privacy policy|Modern Slavery Act Transparency Statement|Sitemap|About US| Contact US: help@patsnap.com