Single Frame Super-resolution Reconstruction Method Based on Small Convolutional Recurrent Neural Network
A recurrent neural network and neural network technology, applied in the field of single-frame super-resolution reconstruction, can solve problems such as high cost, high computational complexity, and inability to apply imaging environments, and achieve the effect of improving operating efficiency
- Summary
- Abstract
- Description
- Claims
- Application Information
AI Technical Summary
Problems solved by technology
Method used
Image
Examples
Embodiment Construction
[0042] The present invention will be described in further detail below in conjunction with the accompanying drawings and specific embodiments.
[0043] The main problem to be solved by the present invention is to provide a single-frame super-resolution reconstruction method based on a small convolutional recurrent neural network, which generates a corresponding high-resolution image for a low-resolution image. In order to solve the above-mentioned technical problems, the technical solution adopted by the present invention is: establish a recursive convolutional neural network with a linear activation function, the input of which is a small neighborhood of a certain pixel of the image, and the output is the corresponding temporary output result of the pixel position; Using the recursive convolutional neural network established in the previous step, input a low-resolution image, and after a certain number of iterations, output the final super-resolution imaging result. Specifica...
PUM
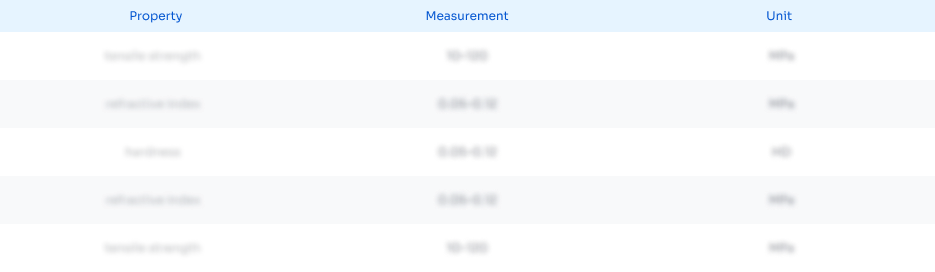
Abstract
Description
Claims
Application Information
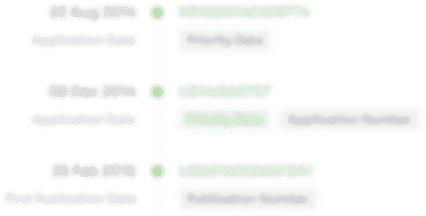
- R&D Engineer
- R&D Manager
- IP Professional
- Industry Leading Data Capabilities
- Powerful AI technology
- Patent DNA Extraction
Browse by: Latest US Patents, China's latest patents, Technical Efficacy Thesaurus, Application Domain, Technology Topic, Popular Technical Reports.
© 2024 PatSnap. All rights reserved.Legal|Privacy policy|Modern Slavery Act Transparency Statement|Sitemap|About US| Contact US: help@patsnap.com