Inter-carrier interference resistant OFDM detection method based on deep learning
A technology of inter-subcarrier interference and deep learning, applied in the direction of modulated carrier system, phase-modulated carrier system, baseband system, etc., to achieve the effect of improving system performance
- Summary
- Abstract
- Description
- Claims
- Application Information
AI Technical Summary
Problems solved by technology
Method used
Image
Examples
example 1
[0067] The simulation conditions are as follows:
[0068] Number of subcarriers
[0069] The simulation channel uses the Rayleigh channel with six delay paths, and the relative power level uses the ITU Vehicle-A channel model parameters. figure 2 The simulation results under the above conditions are given. It can be seen that compared with the direct decorrelation method, the bit error rate of deep network detection is about 2dB lower.
example 2
[0071] The simulation conditions are as follows:
[0072] Number of subcarriers
[0073] The simulation channel uses the Rayleigh channel with six delay paths, and the relative power level uses the ITU Vehicle-A channel model parameters. image 3 The simulation results under the above conditions are given. It can be seen that compared with the direct decorrelation method, the bit error rate of deep network detection is about 4dB lower. and figure 2 In comparison, it can also be seen that the performance of the deep detection network is basically not affected by the size of the Doppler frequency offset.
example 3
[0075] The simulation conditions are as follows:
[0076] Number of subcarriers
[0077] The simulated channel uses the Rayleigh channel with four delay paths, and the relative power level uses the ITU Pedestrian-A channel model parameters. Figure 4 The simulation results under the above conditions are given. It can be seen that compared with the direct decorrelation method, the bit error rate of deep network detection is about 4-6dB lower.
PUM
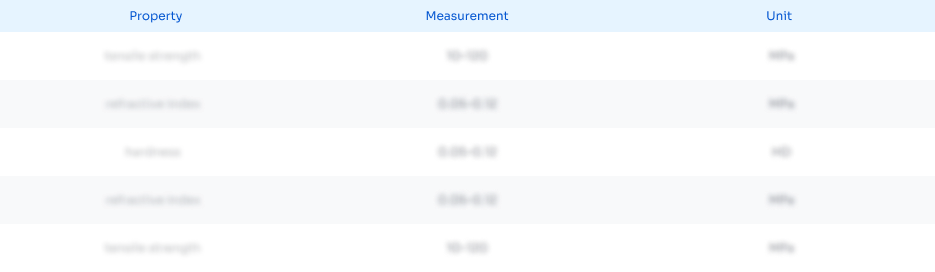
Abstract
Description
Claims
Application Information
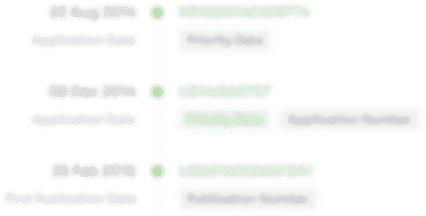
- R&D
- Intellectual Property
- Life Sciences
- Materials
- Tech Scout
- Unparalleled Data Quality
- Higher Quality Content
- 60% Fewer Hallucinations
Browse by: Latest US Patents, China's latest patents, Technical Efficacy Thesaurus, Application Domain, Technology Topic, Popular Technical Reports.
© 2025 PatSnap. All rights reserved.Legal|Privacy policy|Modern Slavery Act Transparency Statement|Sitemap|About US| Contact US: help@patsnap.com