Adversarial-network-based semi-supervised semantic segmentation method
A semantic segmentation and semi-supervised technology, applied in biological neural network models, instruments, character and pattern recognition, etc., can solve problems such as poor semantic segmentation of images
- Summary
- Abstract
- Description
- Claims
- Application Information
AI Technical Summary
Problems solved by technology
Method used
Image
Examples
Embodiment Construction
[0036] It should be noted that, in the case of no conflict, the embodiments in the present application and the features in the embodiments can be combined with each other. The present invention will be further described in detail below in conjunction with the drawings and specific embodiments.
[0037] figure 1 It is a system overview diagram of a semi-supervised semantic segmentation method based on an adversarial network in the present invention. Its main content includes network architecture and training process.
[0038] Wherein, the described network architecture mainly includes:
[0039] (1) Segmentation network: Use the DeepLab-v2 framework with the ResNet-101 model and pre-trained in the ImageNet database as the segmentation network, remove the last classification network layer, and change the distance between the last two convolutional layers from 2 to 1; this makes the resolution of the output image one-eighth the size of the input image;
[0040] In order to incr...
PUM
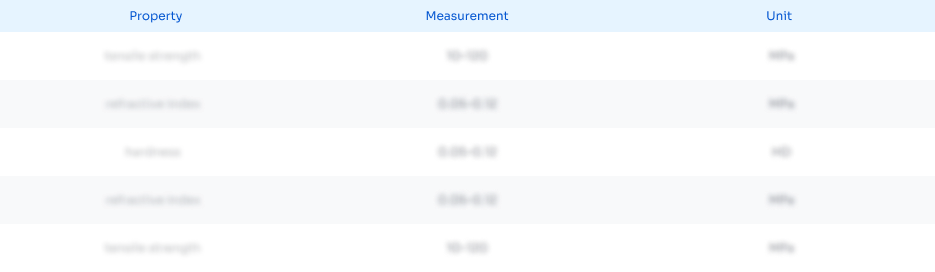
Abstract
Description
Claims
Application Information
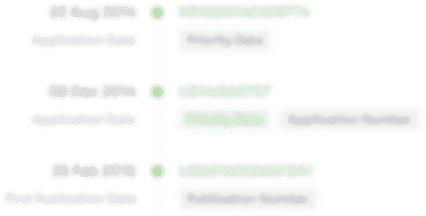
- R&D Engineer
- R&D Manager
- IP Professional
- Industry Leading Data Capabilities
- Powerful AI technology
- Patent DNA Extraction
Browse by: Latest US Patents, China's latest patents, Technical Efficacy Thesaurus, Application Domain, Technology Topic, Popular Technical Reports.
© 2024 PatSnap. All rights reserved.Legal|Privacy policy|Modern Slavery Act Transparency Statement|Sitemap|About US| Contact US: help@patsnap.com