Complex visual image reconstruction method based on depth encoding and decoding dual model
A dual model and visual image technology, applied in the field of visual scene reconstruction, can solve problems such as long reconstruction time, large noise, and low image accuracy
- Summary
- Abstract
- Description
- Claims
- Application Information
AI Technical Summary
Problems solved by technology
Method used
Image
Examples
Embodiment Construction
[0042] A. Coding model:
[0043] Step A1: Perform padding operation on the original natural stimulus image with a size of 256*256*3 to obtain data with a size of 262*262*3. Then three operations are performed on the zero-padded data in sequence, and each operation includes three operations: Convolution (convolution), Batch Normalization (batch normalization), and Relu (corrected linear unit nonlinear function). The convolution kernel sizes of the convolution operations in the three operations are: 7*7, 3*3, 3*3; the convolution steps are 1, 2, and 2; the convolution kernel depths are 64, 128, and 256, respectively. This step finally results in data with a size of 64*64*256.
[0044] Step A2: Use the data with a size of 64*64*256 finally obtained in step A1 as the input of this step, and perform 9 Residual operations on it. Each residual operation does not change the size (first two dimensions) and thickness (third dimension) of the data. So the final size of this step is st...
PUM
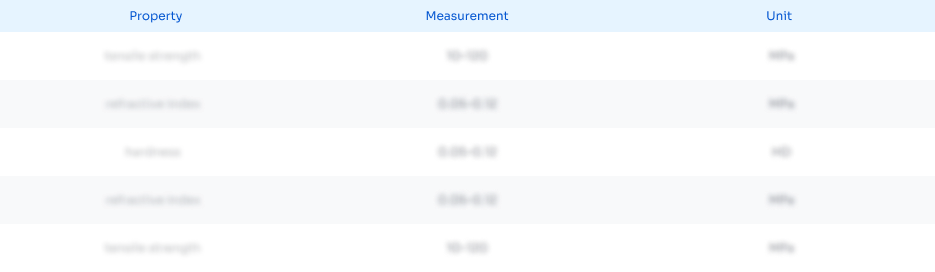
Abstract
Description
Claims
Application Information
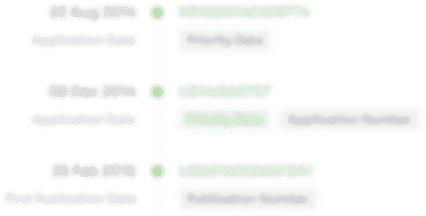
- Generate Ideas
- Intellectual Property
- Life Sciences
- Materials
- Tech Scout
- Unparalleled Data Quality
- Higher Quality Content
- 60% Fewer Hallucinations
Browse by: Latest US Patents, China's latest patents, Technical Efficacy Thesaurus, Application Domain, Technology Topic, Popular Technical Reports.
© 2025 PatSnap. All rights reserved.Legal|Privacy policy|Modern Slavery Act Transparency Statement|Sitemap|About US| Contact US: help@patsnap.com