Human face recognition method and device based on residual-quantized convolutional neural network
A convolutional neural network and face recognition technology, which is applied to face recognition methods and devices, and the field of face recognition methods and devices based on residual quantization convolutional neural networks, can solve massive calculations, high overhead, and improve hardware requirements. and other problems, to achieve the effect of speeding up feature extraction and shortening computing time.
- Summary
- Abstract
- Description
- Claims
- Application Information
AI Technical Summary
Problems solved by technology
Method used
Image
Examples
Embodiment Construction
[0028] The specific implementation manners of the present invention will be described below in conjunction with the drawings and embodiments.
[0029]
[0030] The model construction and the like in this embodiment are all implemented on the Linux platform, which has the support of at least one graphics processing unit (GPU) card.
[0031] figure 1 It is a flow chart of the face recognition method based on residual quantization convolutional neural network according to the embodiment of the present invention.
[0032] Such as figure 1 As shown, the face recognition method based on residual quantization convolutional neural network mainly includes the following steps.
[0033] Step S1, model construction and training. That is, construct a convolutional neural network model and use multiple existing face images as a training set to perform training on the convolutional neural network model based on residual quantization, and the obtained trained convolutional neural network...
PUM
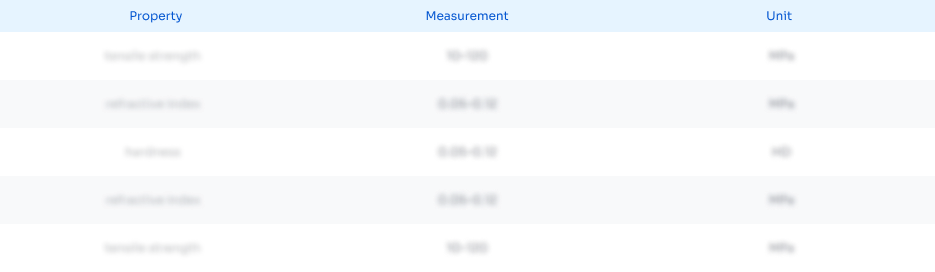
Abstract
Description
Claims
Application Information
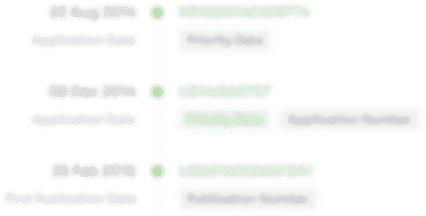
- R&D Engineer
- R&D Manager
- IP Professional
- Industry Leading Data Capabilities
- Powerful AI technology
- Patent DNA Extraction
Browse by: Latest US Patents, China's latest patents, Technical Efficacy Thesaurus, Application Domain, Technology Topic, Popular Technical Reports.
© 2024 PatSnap. All rights reserved.Legal|Privacy policy|Modern Slavery Act Transparency Statement|Sitemap|About US| Contact US: help@patsnap.com